Introduction
Note
|
Go here for documentation for APOC version 3.1.x documentation for APOC version 3.2.x |
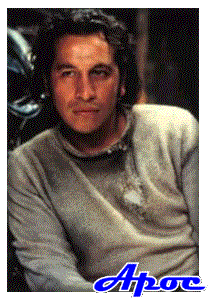
Neo4j 3.0 introduced the concept of user defined procedures. Those are custom implementations of certain functionality, that can’t be (easily) expressed in Cypher itself. Those procedures are implemented in Java and can be easily deployed into your Neo4j instance, and then be called from Cypher directly.
The APOC library consists of many (about 300) procedures to help with many different tasks in areas like data integration, graph algorithms or data conversion.
License
Apache License 2.0
"APOC" Name history
Apoc was the technician and driver on board of the Nebuchadnezzar in the Matrix movie. He was killed by Cypher.
APOC was also the first bundled A Package Of Components for Neo4j in 2009.
APOC also stands for "Awesome Procedures On Cypher"
Installation
Download latest release
to find the latest release and download the binary jar to place into your $NEO4J_HOME/plugins
folder.
Version Compatibility Matrix
Since APOC relies in some places on Neo4j’s internal APIs you need to use the right APOC version for your Neo4j installaton.
Any version to be released after 1.1.0 will use a different, consistent versioning scheme: <neo4j-version>.<apoc>
version. The trailing <apoc>
part of the version number will be incremented with every apoc release.
apoc version |
neo4j version |
3.2.0-alpha07 (3.2.x) |
|
3.1.3 (3.1.x) |
|
3.1.2 |
|
3.1.0-3.1.1 |
|
3.0.5-3.0.9 (3.0.x) |
|
3.0.4.3 |
3.0.4 |
1.1.0 |
3.0.0 - 3.0.3 |
1.0.0 |
3.0.0 - 3.0.3 |
using APOC with Neo4j Docker image
The Neo4j Docker image allows to supply a volume for the /plugins
folder. Download the APOC release fitting your Neo4j version to local folder plugins
and provide it as a data volume:
mkdir plugins
pushd plugins
wget https://github.com/neo4j-contrib/neo4j-apoc-procedures/releases/download/3.0.8.6/apoc-3.0.8.6-all.jar
popd
docker run --rm -e NEO4J_AUTH=none -p 7474:7474 -v $PWD/plugins:/plugins -p 7687:7687 neo4j:3.0.9
Build & install the current development branch from source
git clone http://github.com/neo4j-contrib/neo4j-apoc-procedures cd neo4j-apoc-procedures ./gradlew shadow cp build/libs/apoc-<version>-SNAPSHOT-all.jar $NEO4J_HOME/plugins/ $NEO4J_HOME/bin/neo4j restart
A full build including running the tests can be run by ./gradlew build
.
Calling Procedures & Functions within Cypher
User defined Functions can be used in any expression or predicate, just like built-in functions.
Procedures can be called stand-alone with CALL procedure.name();
But you can also integrate them into your Cypher statements which makes them so much more powerful.
WITH 'https://raw.githubusercontent.com/neo4j-contrib/neo4j-apoc-procedures/master/src/test/resources/person.json' AS url
CALL apoc.load.json(url) YIELD value as person
MERGE (p:Person {name:person.name})
ON CREATE SET p.age = person.age, p.children = size(person.children)
Procedure & Function Signatures
To call procedures correctly, you need to know their parameter names, types and positions. And for YIELDing their results the output column name and type.
You can see the procedures signature in the output of CALL dbms.procedures()
(The same applies for functions with CALL dbms.functions()
)
CALL dbms.procedures() YIELD name, signature
WITH * WHERE name STARTS WITH 'apoc.algo.dijkstra'
RETURN name, signature
The signature is always name : : TYPE
, so in this case:
apoc.algo.dijkstra (startNode :: NODE?, endNode :: NODE?, relationshipTypesAndDirections :: STRING?, weightPropertyName :: STRING?) :: (path :: PATH?, weight :: FLOAT?)
Parameters:
Name | Type |
---|---|
Procedure Parameters |
|
|
|
|
|
|
|
|
|
Output Return Columns |
|
|
|
|
|
Help and Usage
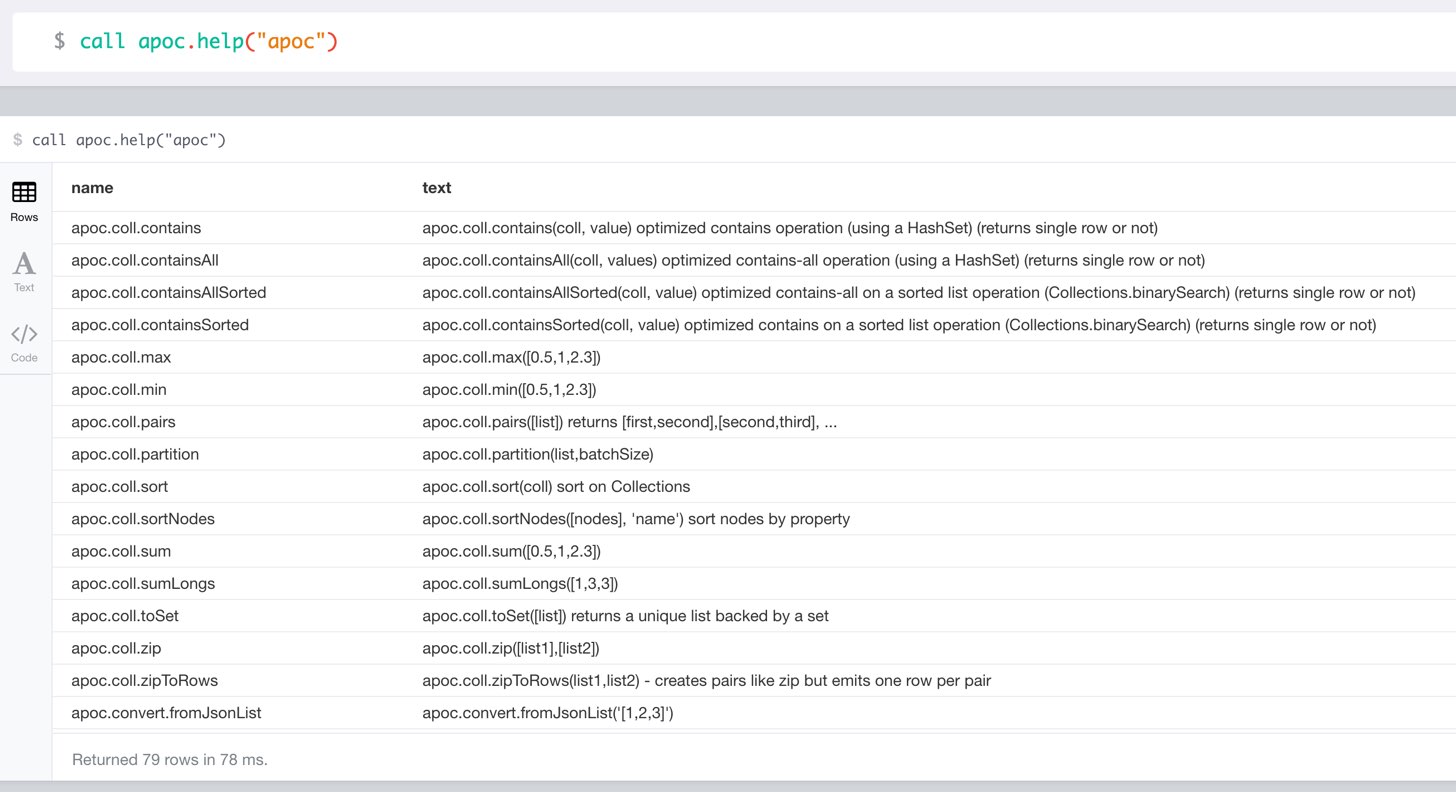
|
lists name, description-text and if the procedure performs writes, search string is checked against beginning (package) or end (name) of procedure |
CALL apoc.help("apoc") YIELD name, text
WITH * WHERE text IS null
RETURN name AS undocumented
To generate the help from @Description
annotations, apoc currently scans the jar file with ASM.
Overview of APOC Procedures
Configuration Options
Set these config options in $NEO4J_HOME/neo4j.conf
All boolean options default to false, i.e. are disabled, unless mentioned otherwise.
|
Enable triggers |
|
Enable time to live background task |
|
Set frequency in seconds to run ttl background task (default 60) |
|
Enable reading local files from disk |
|
Enable writing local files to disk |
|
store jdbc-urls under a key to be used by apoc.load.jdbc |
|
store es-urls under a key to be used by elasticsearch procedures |
|
store mongodb-urls under a key to be used by mongodb procedures |
|
store couchbase-urls under a key to be used by couchbase procedures |
Manual Indexes
Index Queries
Procedures to add to and query manual indexes
Note
|
Please note that there are (case-sensitive) automatic schema indexes, for equality, non-equality, existence, range queries, starts with, ends-with and contains! |
|
add all nodes to this full text index with the given fields, additionally populates a 'search' index field with all of them in one place |
|
add node to an index for each label it has |
|
add node to an index for the given label |
|
add node to an index for the given name |
|
add relationship to an index for its type |
|
add relationship to an index for the given name |
|
apoc.index.removeRelationshipByName('name',rel) remove relationship from an index for the given name |
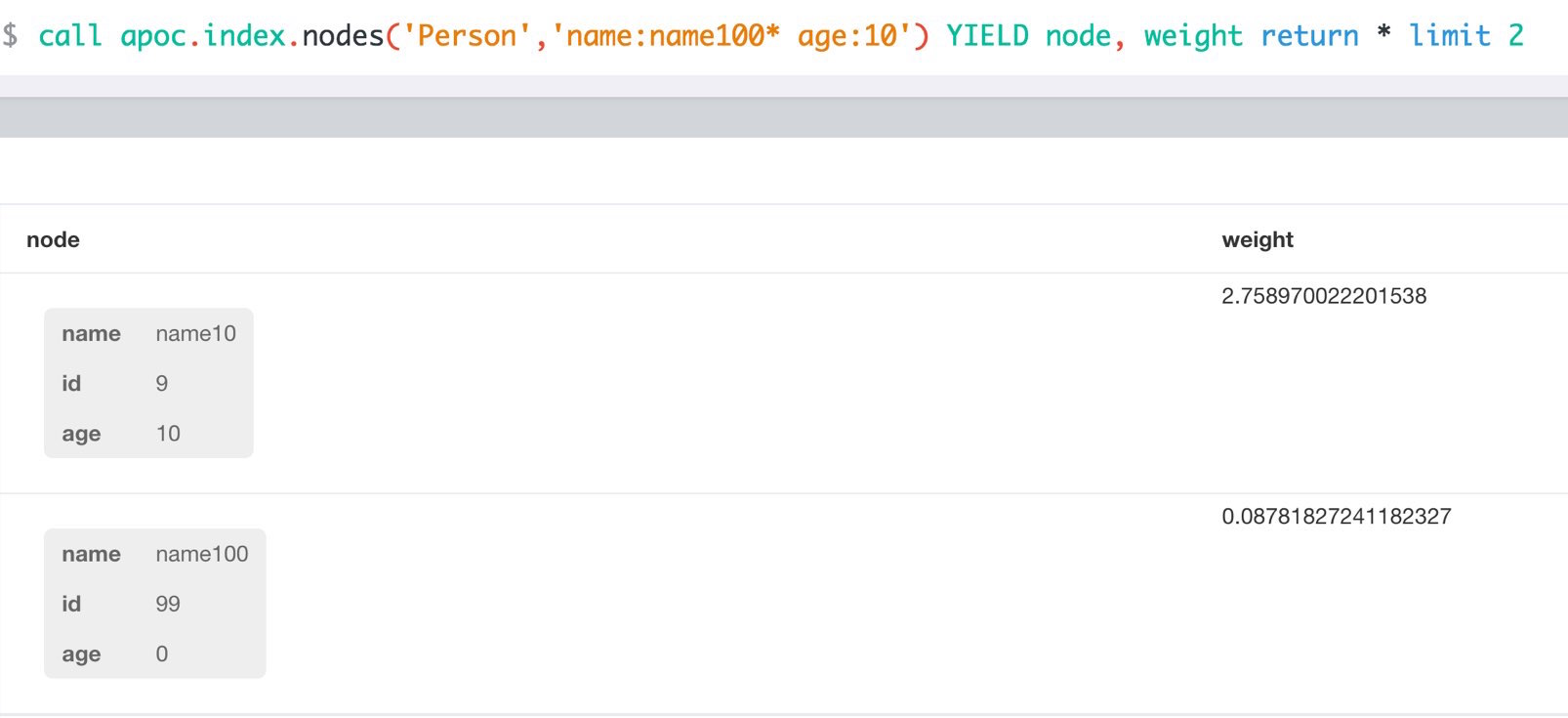
|
search for the first 100 nodes in the given full text index matching the given lucene query returned by relevance |
|
lucene query on node index with the given label name |
|
lucene query on relationship index with the given type name |
|
lucene query on relationship index with the given type name bound by either or both sides (each node parameter can be null) |
|
lucene query on relationship index with the given type name for outgoing relationship of the given node, returns end-nodes |
|
lucene query on relationship index with the given type name for incoming relationship of the given node, returns start-nodes |
Index Management
|
lists all manual indexes |
|
removes manual indexes |
|
gets or creates manual node index |
|
gets or creates manual relationship index |
match (p:Person) call apoc.index.addNode(p,["name","age"]) RETURN count(*);
// 129s for 1M People
call apoc.index.nodes('Person','name:name100*') YIELD node, weight return * limit 2
Schema Index Queries
Schema Index lookups that keep order and can apply limits
|
schema range scan which keeps index order and adds limit, values can be null, boundaries are inclusive |
|
schema string search which keeps index order and adds limit, operator is 'STARTS WITH' or 'CONTAINS' |
Meta Graph
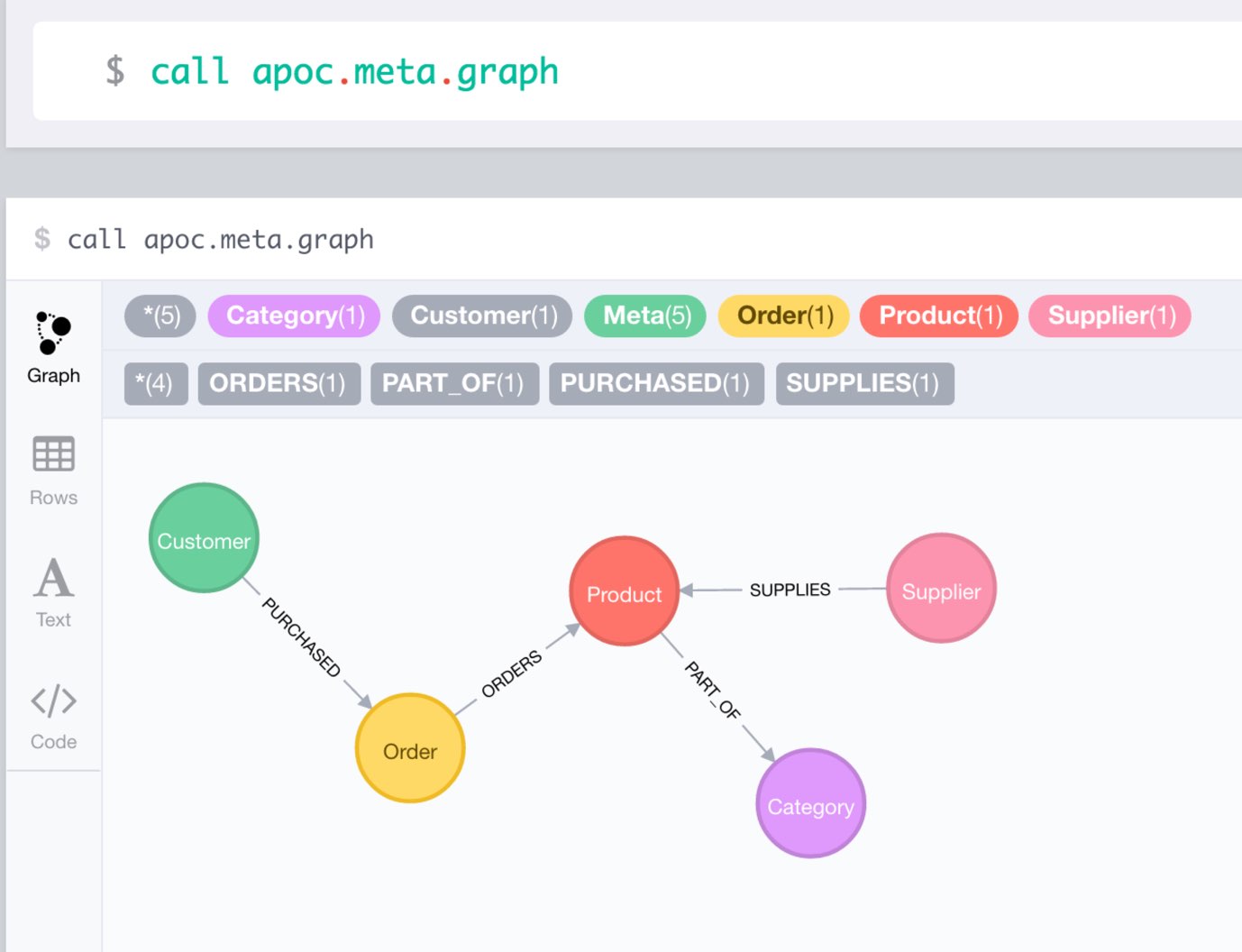
Returns a virtual graph that represents the labels and relationship-types available in your database and how they are connected.
|
examines the database statistics to build the meta graph, very fast, might report extra relationships |
|
examines the database statistics to create the meta-graph, post filters extra relationships by sampling |
|
examines a sample sub graph to create the meta-graph |
|
examines a subset of the graph to provide a tabular meta information |
|
returns the information stored in the transactional database statistics |
|
type name of a value ( |
|
returns a row if type name matches none if not |
|
returns a a map of property-keys to their names |
MATCH (n:Person)
CALL apoc.meta.isType(n.age,"INTEGER")
RETURN n LIMIT 5
Schema
|
asserts that at the end of the operation the given indexes and unique constraints are there, each label:key pair is considered one constraint/label |
Locking
|
acquires a write lock on the given nodes |
|
acquires a write lock on the given relationship |
|
acquires a write lock on the given nodes and relationships |
from/toJson
|
converts value to json string |
|
converts value to json map |
|
converts json list to Cypher list |
|
converts json map to Cypher map |
|
sets value serialized to JSON as property with the given name on the node |
|
converts serialized JSON in property back to original object |
|
converts serialized JSON in property back to map |
|
creates a stream of nested documents representing the at least one root of these paths |
Export / Import
Export to CSV
YIELD file, source, format, nodes, relationships, properties, time, rows
|
exports results from the cypher statement as csv to the provided file |
|
exports whole database as csv to the provided file |
|
exports given nodes and relationships as csv to the provided file |
|
exports given graph object as csv to the provided file |
Export to Cypher Script
Data is exported as cypher statements (for neo4j-shell, and partly apoc.cypher.runFile to the given file.
YIELD file, source, format, nodes, relationships, properties, time
|
exports whole database incl. indexes as cypher statements to the provided file |
|
exports given nodes and relationships incl. indexes as cypher statements to the provided file |
|
exports given graph object incl. indexes as cypher statements to the provided file |
|
exports nodes and relationships from the cypher statement incl. indexes as cypher statements to the provided file |
GraphML Import / Export
GraphML is used by other tools, like Gephi and CytoScape to read graph data.
YIELD file, source, format, nodes, relationships, properties, time
|
imports graphml into the graph |
|
exports whole database as graphml to the provided file |
|
exports given nodes and relationships as graphml to the provided file |
|
exports given graph object as graphml to the provided file |
|
exports nodes and relationships from the cypher statement as graphml to the provided file |
Loading Data from RDBMS
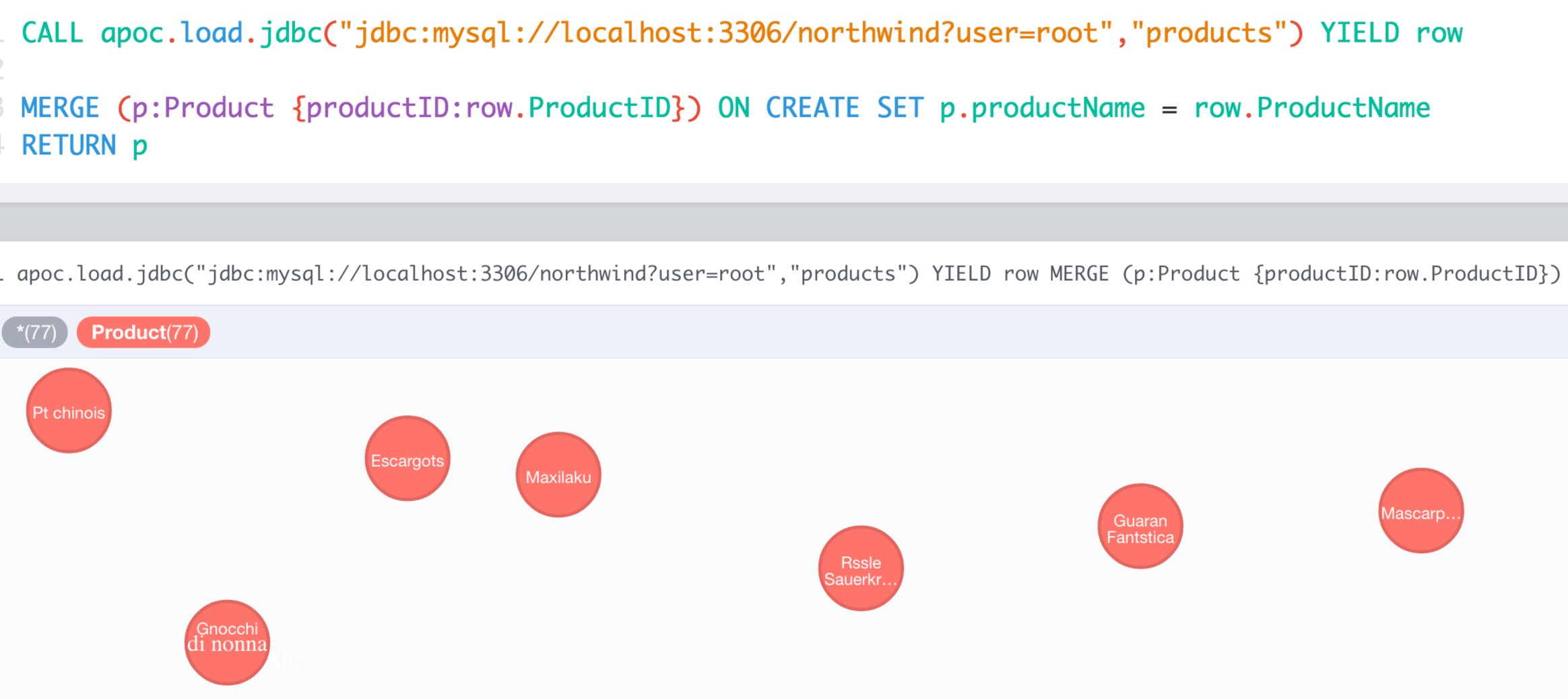
|
load from relational database, either a full table or a sql statement |
|
load from relational database, either a full table or a sql statement |
|
register JDBC driver of source database |
To simplify the JDBC URL syntax and protect credentials, you can configure aliases in conf/neo4j.conf
:
apoc.jdbc.myDB.url=jdbc:derby:derbyDB
CALL apoc.load.jdbc('jdbc:derby:derbyDB','PERSON') becomes CALL apoc.load.jdbc('myDB','PERSON')
The 3rd value in the apoc.jdbc.<alias>.url=
effectively defines an alias to be used in apoc.load.jdbc('<alias>',….
Loading Data from Web-APIs (JSON, XML, CSV)
|
load from JSON URL (e.g. web-api) to import JSON as stream of values if the JSON was an array or a single value if it was a map |
|
load from XML URL (e.g. web-api) to import XML as single nested map with attributes and |
|
load from XML URL (e.g. web-api) to import XML as single nested map with attributes and |
|
load CSV fom URL as stream of values |
Interacting with Elastic Search
|
elastic search statistics |
|
perform a GET operation |
|
perform a SEARCH operation |
|
perform a raw GET operation |
|
perform a raw POST operation |
|
perform a POST operation |
|
perform a PUT operation |
Interacting with MongoDB
|
perform a find operation on mongodb collection |
|
perform a find operation on mongodb collection |
|
perform a first operation on mongodb collection |
|
perform a find,project,sort operation on mongodb collection |
|
inserts the given documents into the mongodb collection |
|
inserts the given documents into the mongodb collection |
|
inserts the given documents into the mongodb collection |
Copy these jars into the plugins directory:
mvn dependency:copy-dependencies cp target/dependency/mongodb*.jar target/dependency/bson*.jar $NEO4J_HOME/plugins/
CALL apoc.mongodb.first('mongodb://localhost:27017','test','test',{name:'testDocument'})
Interacting with Couchbase
|
Retrieves a couchbase json document by its unique ID |
|
Check whether a couchbase json document with the given ID does exist |
|
Insert a couchbase json document with its unique ID |
|
Insert or overwrite a couchbase json document with its unique ID |
|
Append a couchbase json document to an existing one |
|
Prepend a couchbase json document to an existing one |
|
Remove the couchbase json document identified by its unique ID |
|
Replace the content of the couchbase json document identified by its unique ID. |
|
Executes a plain un-parameterized N1QL statement. |
|
Executes a N1QL statement with positional parameters. |
|
Executes a N1QL statement with named parameters. |
Copy these jars into the plugins directory:
mvn dependency:copy-dependencies cp target/dependency/java-client-2.3.1.jar target/dependency/core-io-1.3.1.jar target/dependency/rxjava-1.1.5.jar $NEO4J_HOME/plugins/
CALL apoc.couchbase.get(['localhost'], 'default', 'artist:vincent_van_gogh')
Streaming Data to Gephi
|
streams provided data to Gephi |
Notes
Gephi has a streaming plugin, that can provide and accept JSON-graph-data in a streaming fashion.
Make sure to install the plugin firsrt and activate it for your workspace (there is a new "Streaming"-tab besides "Layout"), right-click "Master"→"start" to start the server.
You can provide your workspace name (you might want to rename it before you start thes streaming), otherwise it defaults to workspace0
The default Gephi-URL is http://localhost:8080, resulting in http://localhost:8080/workspace0?operation=updateGraph
You can also configure it in conf/neo4j.conf
via apoc.gephi.url=url
or apoc.gephi.<key>.url=url
Example
match path = (:Person)-[:ACTED_IN]->(:Movie)
WITH path LIMIT 1000
with collect(path) as paths
call apoc.gephi.add(null,'workspace0', paths) yield nodes, relationships, time
return nodes, relationships, time
Creating Data
|
create node with dynamic labels |
|
create multiple nodes with dynamic labels |
|
adds the given labels to the node or nodes |
|
removes the given labels from the node or nodes |
|
create relationship with dynamic rel-type |
|
creates an UUID |
|
creates count UUIDs |
|
creates a linked list of nodes from first to last |
|
returns each node and a 'dense' flag if it is a dense node |
|
yields true effectively when the node has the relationships of the pattern |
Virtual Nodes/Rels
Virtual Nodes and Relationships don’t exist in the graph, they are only returned to the UI/user for representing a graph projection. They can be visualized or processed otherwise. Please note that they have negative id’s.
|
returns a virtual node |
|
returns virtual nodes |
|
returns a virtual relationship |
|
returns a virtual pattern |
|
returns a virtual pattern |
Example
MATCH (a)-[r]->(b)
WITH head(labels(a)) AS l, head(labels(b)) AS l2, type(r) AS rel_type, count(*) as count
CALL apoc.create.vNode(['Meta_Node'],{name:l}) yield node as a
CALL apoc.create.vNode(['Meta_Node'],{name:l2}) yield node as b
CALL apoc.create.vRelationship(a,'META_RELATIONSHIP',{name:rel_type, count:count},b) yield rel
RETURN *;
Virtual Graph
Create a graph object (map) from information that’s passed in.
It’s basic structure is: {name:"Name",properties:{properties},nodes:[nodes],relationships:[relationships]}
|
creates a virtual graph object for later processing it tries its best to extract the graph information from the data you pass in |
|
creates a virtual graph object for later processing |
|
creates a virtual graph object for later processing |
|
creates a virtual graph object for later processing |
|
creates a virtual graph object for later processing |
|
creates a virtual graph object for later processing |
Generating Graphs
Generate undirected (random direction) graphs with semi-real random distributions based on theoretical models.
|
generates a graph according to Erdos-Renyi model (uniform) |
|
generates a graph according to Watts-Strogatz model (clusters) |
|
generates a graph according to Barabasi-Albert model (preferential attachment) |
|
generates a complete graph (all nodes connected to all other nodes) |
|
generates a graph with the given degree distribution |
Example
CALL apoc.generate.ba(1000, 2, 'TestLabel', 'TEST_REL_TYPE')
CALL apoc.generate.ws(1000, null, null, null)
CALL apoc.generate.simple([2,2,2,2], null, null)
Warmup
(thanks @SaschaPeukert)
|
Warmup the node and relationship page-caches by loading one page at a time |
Monitoring
(thanks @ikwattro)
|
node and relationships-ids in total and in use |
|
store information such as kernel version, start time, read-only, database-name, store-log-version etc. |
|
store size information for the different types of stores |
|
number of transactions total,opened,committed,concurrent,rolled-back,last-tx-id |
|
db locking information such as avertedDeadLocks, lockCount, contendedLockCount and contendedLocks etc. (enterprise) |
Cypher Execution
|
executes reading fragment with the given parameters |
|
runs each statement in the file, all semicolon separated - currently no schema operations |
|
runs each semicolon separated statement and returns summary - currently no schema operations |
|
executes fragment in parallel batches with the list segments being assigned to _ |
|
executes writing fragment with the given parameters |
|
abort statement after timeout millis if not finished |
Triggers
Enable apoc.trigger.enabled=true
in $NEO4J_HOME/config/neo4j.conf
first.
|
add a trigger statement under a name, in the statement you can use {createdNodes}, {deletedNodes} etc., the selector is {phase:'before/after/rollback'} returns previous and new trigger information |
|
remove previously added trigger, returns trigger information |
|
update and list all installed triggers |
CALL apoc.trigger.add('timestamp','UNWIND {createdNodes} AS n SET n.ts = timestamp()');
CALL apoc.trigger.add('lowercase','UNWIND {createdNodes} AS n SET n.id = toLower(n.name)');
CALL apoc.trigger.add('txInfo', 'UNWIND {createdNodes} AS n SET n.txId = {transactionId}, n.txTime = {commitTime}', {phase:'after'});
CALL apoc.trigger.add('count-removed-rels','MATCH (c:Counter) SET c.count = c.count + size([r IN {deletedRelationships} WHERE type(r) = "X"])')
Job Management
|
repeats an batch update statement until it returns 0, this procedure is blocking |
|
list all jobs |
|
submit a one-off background statement |
|
submit a repeatedly-called background statement |
|
submit a repeatedly-called background statement until it returns 0 |
|
iterate over first statement and apply action statement with given transaction batch size. Returns to numeric values holding the number of batches and the number of total processed rows. E.g. |
|
run the second statement for each item returned by the first statement. Returns number of batches and total processed rows |
-
there are also static methods
Jobs.submit
, andJobs.schedule
to be used from other procedures -
jobs list is checked / cleared every 10s for finished jobs
name
property of each person to lastname
CALL apoc.periodic.rock_n_roll('match (p:Person) return id(p) as id_p', 'MATCH (p) where id(p)={id_p} SET p.lastname =p.name', 20000)
Graph Refactoring
|
clone nodes with their labels and properties |
|
clone nodes with their labels, properties and relationships |
|
merge nodes onto first in list |
|
redirect relationship to use new end-node |
|
redirect relationship to use new start-node |
|
inverts relationship direction |
|
change relationship-type |
|
extract node from relationships |
|
collapse node to relationship, node with one rel becomes self-relationship |
|
normalize/convert a property to be boolean |
|
turn each unique propertyKey into a category node and connect to it |
TODO:
-
merge nodes by label + property
-
merge relationships
Spatial
|
look up geographic location of location from openstreetmap geocoding service |
|
sort a given collection of paths by geographic distance based on lat/long properties on the path nodes |
Helpers
Static Value Storage
|
returns statically stored value from config (apoc.static.<key>) or server lifetime storage |
|
returns statically stored values from config (apoc.static.<prefix>) or server lifetime storage |
|
stores value under key for server livetime storage, returns previously stored or configured value |
Conversion Functions
Sometimes type information gets lost, these functions help you to coerce an "Any" value to the concrete type
|
tries it’s best to convert the value to a string |
|
tries it’s best to convert the value to a map |
|
tries it’s best to convert the value to a list |
|
tries it’s best to convert the value to a boolean |
|
tries it’s best to convert the value to a node |
|
tries it’s best to convert the value to a relationship |
|
tries it’s best to convert the value to a set |
Map Functions
|
creates map from nodes with this label grouped by property |
|
creates map from list with key-value pairs |
|
creates map from a keys and a values list |
|
creates map from alternating keys and values in a list |
|
creates map from merging the two source maps |
|
merges all maps in the list into one |
|
returns the map with the value for this key added or replaced |
|
returns the map with the key removed |
|
returns the map with the keys removed |
|
removes the keys and values (e.g. null-placeholders) contained in those lists, good for data cleaning from CSV/JSON |
|
creates a map of the list keyed by the given property, with single values |
|
creates a map of the list keyed by the given property, with list values |
Collection Functions
|
sum of all values in a list |
|
avg of all values in a list |
|
minimum of all values in a list |
|
maximum of all values in a list |
|
sums all numeric values in a list |
|
partitions a list into sublists of |
|
all values in a list |
|
[1,2],[2,3],[3,null] |
|
[1,2],[2,3] |
|
returns a unique list backed by a set |
|
sort on Collections |
|
sort nodes by property |
|
optimized contains operation (using a HashSet) (returns single row or not) |
|
optimized contains-all operation (using a HashSet) (returns single row or not) |
|
optimized contains on a sorted list operation (Collections.binarySearch) (returns single row or not) |
|
optimized contains-all on a sorted list operation (Collections.binarySearch) (returns single row or not) |
|
creates the distinct union of the 2 lists |
|
returns unique set of first list with all elements of second list removed |
|
returns first list with all elements of second list removed |
|
returns the unique intersection of the two lists |
|
returns the disjunct set of the two lists |
|
creates the full union with duplicates of the two lists |
|
splits collection on given values rows of lists, value itself will not be part of resulting lists |
|
position of value in the list |
Lookup Functions
|
id |
|
quickly returns all nodes with these id’s |
|
id |
|
quickly returns all relationships with these id’s |
Text Functions
|
replace each substring of the given string that matches the given regular expression with the given replacement. |
|
join the given strings with the given delimiter. |
|
sprintf format the string with the params given |
|
left pad the string to the given width |
|
right pad the string to the given width |
|
returns domain part of the value |
Phonetic Comparisons
|
Compute the US_ENGLISH phonetic soundex encoding of all words of the text value which can be a single string or a list of strings |
|
Compute the US_ENGLISH soundex character difference between two given strings |
|
strip the given string of everything except alpha numeric characters and convert it to lower case. |
|
compare the given strings stripped of everything except alpha numeric characters converted to lower case. |
|
filter out non-matches of the given strings stripped of everything except alpha numeric characters converted to lower case. |
Utilities
|
computes the sha1 of the concatenation of all string values of the list |
|
computes the md5 of the concatenation of all string values of the list |
|
sleeps for <duration> millis, transaction termination is honored |
|
raises exception if prediate evaluates to true |
Config
|
Lists the Neo4j configuration as key,value table |
|
Lists the Neo4j configuration as map |
Time to Live (TTL)
Enable TTL with setting in neo4j.conf
: apoc.ttl.enabled=true
There are some convenience procedures to expire nodes.
You can also do it yourself by running
SET n:TTL
SET n.ttl = timestamp() + 3600
|
expire node in given time-delta by setting :TTL label and |
|
expire node at given time by setting :TTL label and |
Optionally set apoc.ttl.schedule=5
as repeat frequency.
Date/time Support
(thanks @tkroman)
Conversion between formatted dates and timestamps
|
get Unix time equivalent of given date (in seconds) |
|
same as previous, but accepts custom datetime format |
|
get string representation of date corresponding to given Unix time (in seconds) |
|
the same as previous, but accepts custom datetime format |
|
return the system timezone default name |
|
get Unix time equivalent of given date (in milliseconds) |
|
same as previous, but accepts custom datetime format |
|
get string representation of date corresponding to given time in milliseconds in UTC time zone |
|
the same as previous, but accepts custom datetime format |
|
the same as previous, but accepts custom time zone |
-
possible unit values:
ms,s,m,h,d
and their long formsmillis,milliseconds,seconds,minutes,hours,days
. -
possible time zone values: Either an abbreviation such as
PST
, a full name such asAmerica/Los_Angeles
, or a custom ID such asGMT-8:00
. Full names are recommended. You can view a list of full names in this Wikipedia page.
Reading separate datetime fields
Splits date (optionally, using given custom format) into fields returning a map from field name to its value.
-
apoc.date.fields('2015-03-25 03:15:59')
-
apoc.date.fieldsFormatted('2015-01-02 03:04:05 EET', 'yyyy-MM-dd HH:mm:ss zzz')
Bitwise operations
Provides a wrapper around the java bitwise operations.
call apoc.bitwise.op(a long, "operation", b long ) yield value as <identifier> |
examples
operator |
name |
example |
result |
a & b |
AND |
call apoc.bitwise.op(60,"&",13) |
12 |
a | b |
OR |
call apoc.bitwise.op(60,"|",13) |
61 |
a ^ b |
XOR |
call apoc.bitwise.op(60,"&",13) |
49 |
~a |
NOT |
call apoc.bitwise.op(60,"&",0) |
-61 |
a << b |
LEFT SHIFT |
call apoc.bitwise.op(60,"<<",2) |
240 |
a >> b |
RIGHT SHIFT |
call apoc.bitwise.op(60,">>",2) |
15 |
a >>> b |
UNSIGNED RIGHT SHIFT |
call apoc.bitwise.op(60,">>>",2) |
15 |
Path Expander
(thanks @keesvegter)
The apoc.path.expand procedure makes it possible to do variable length path traversals where you can specify the direction of the relationship per relationship type and a list of Label names which act as a "whitelist" or a "blacklist". The procedure will return a list of Paths in a variable name called "path".
|
expand from given nodes(s) taking the provided restrictions into account |
Relationship Filter
Syntax: [<]RELATIONSHIP_TYPE1[>]|[<]RELATIONSHIP_TYPE2[>]|…
input | type | direction |
---|---|---|
|
|
Table of Contents
OUTGOING |
|
|
Table of Contents
INCOMING |
|
|
Table of Contents
BOTH |
Label Filter
Syntax: [+-/]LABEL1|LABEL2|…
input | label | result |
---|---|---|
|
|
Table of Contents
include label (whitelist) |
|
|
Table of Contents
exclude label (blacklist) |
|
|
Table of Contents
stop traversal after reaching a friend (but include him) |
Parallel Node Search
Utility to find nodes in parallel (if possible). These procedures return a single list of nodes or a list of 'reduced' records with node id, labels, and the properties where the search was executed upon.
|
A distinct set of Nodes will be returned. |
|
All the found Nodes will be returned. |
|
A merged set of 'minimal' Node information will be returned. One record per node (-id). |
|
All the found 'minimal' Node information will be returned. One record per label and property. |
|
|
(JSON or Map) For every Label-Property combination a search will be executed in parallel (if possible): Label1.propertyOne, label2.propOne and label2.propTwo. |
|
'exact' or 'contains' or 'starts with' or 'ends with' |
Case insensitive string search operators |
|
"<", ">", "=", "<>", "⇐", ">=", "=~" |
Operators |
|
'Keanu' |
The actual search term (string, number, etc). |
CALL apoc.search.nodeAll('{Person: "name",Movie: ["title","tagline"]}','contains','her') YIELD node AS n RETURN n
call apoc.search.nodeReduced({Person: 'born', Movie: ['released']},'>',2000) yield id, labels, properties RETURN *
Graph Algorithms (work in progress)
Provides some graph algorithms (not very optimized yet)
|
run dijkstra with relationship property name as cost function |
|
run dijkstra with relationship property name as cost function and a default weight if the property does not exist |
|
run A* with relationship property name as cost function |
|
run A* with relationship property name as cost function |
|
run allSimplePaths with relationships given and maxNodes |
|
calculate betweenness centrality for given nodes |
|
calculate closeness centrality for given nodes |
|
return relationships between this set of nodes |
|
calculates page rank for given nodes |
|
calculates page rank for given nodes |
|
simple label propagation kernel |
|
search the graph and return all maximal cliques at least at large as the minimum size argument. |
|
search the graph and return all maximal cliques that are at least as large than the minimum size argument and contain this node |
Example: find the weighted shortest path based on relationship property d
from A
to B
following just :ROAD
relationships
MATCH (from:Loc{name:'A'}), (to:Loc{name:'D'})
CALL apoc.algo.dijkstra(from, to, 'ROAD', 'd') yield path as path, weight as weight
RETURN path, weight
MATCH (n:Person)
Text and Lookup Indexes
Index Queries
Procedures to add to and query manual indexes
Note
|
Please note that there are (case-sensitive) automatic schema indexes, for equality, non-equality, existence, range queries, starts with, ends-with and contains! |
|
add all nodes to this full text index with the given fields, additionally populates a 'search' index field with all of them in one place |
|
add node to an index for each label it has |
|
add node to an index for the given label |
|
add node to an index for the given name |
|
add relationship to an index for its type |
|
add relationship to an index for the given name |
|
apoc.index.removeRelationshipByName('name',rel) remove relationship from an index for the given name |
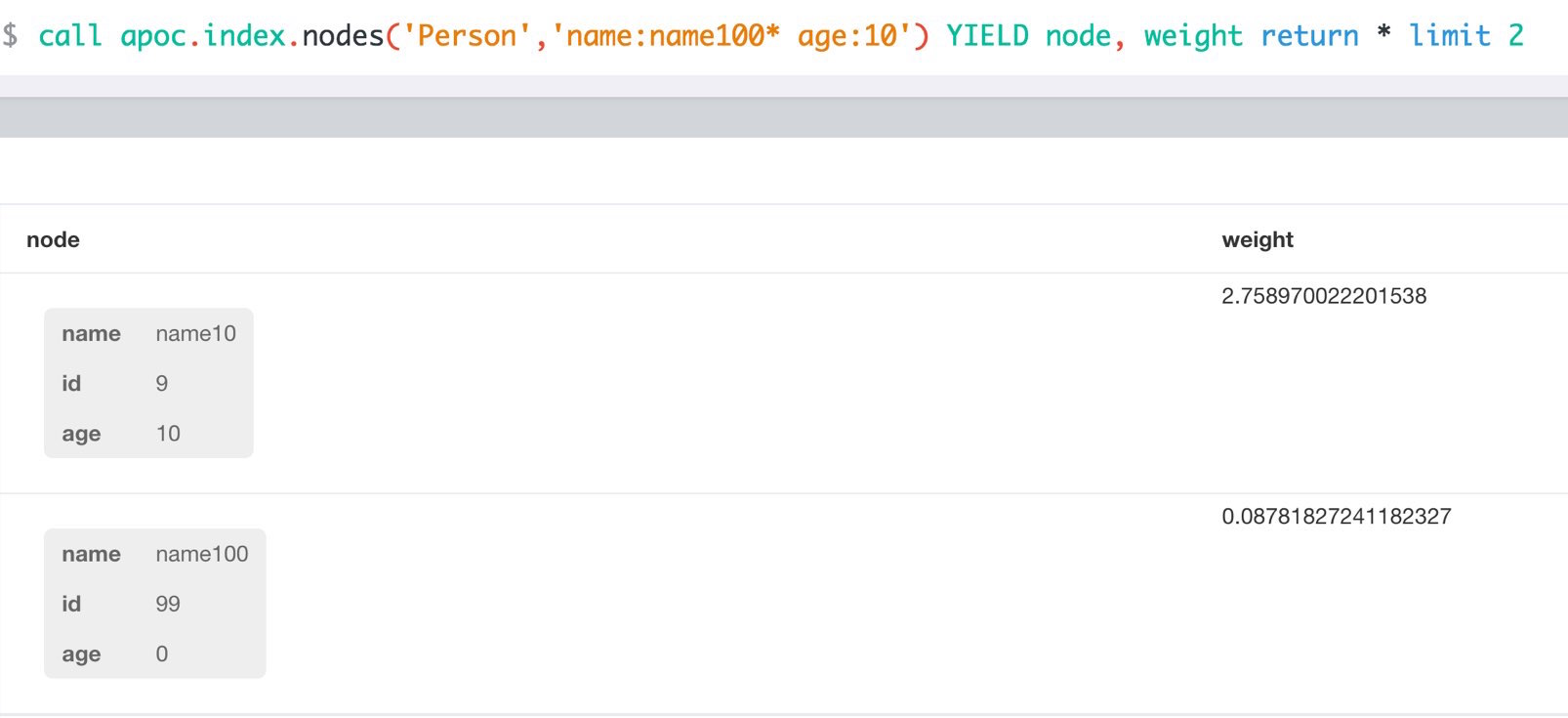
|
search for the first 100 nodes in the given full text index matching the given lucene query returned by relevance |
|
lucene query on node index with the given label name |
|
lucene query on relationship index with the given type name |
|
lucene query on relationship index with the given type name bound by either or both sides (each node parameter can be null) |
|
lucene query on relationship index with the given type name for outgoing relationship of the given node, returns end-nodes |
|
lucene query on relationship index with the given type name for incoming relationship of the given node, returns start-nodes |
Index Management
|
lists all manual indexes |
|
removes manual indexes |
|
gets or creates manual node index |
|
gets or creates manual relationship index |
match (p:Person) call apoc.index.addNode(p,["name","age"]) RETURN count(*);
// 129s for 1M People
call apoc.index.nodes('Person','name:name100*') YIELD node, weight return * limit 2
Manual Indexes
Data Used
The below examples use flight data.
Here is a sample subset of the data that can be load to try the procedures:
CREATE (slc:Airport {abbr:'SLC', id:14869, name:'SALT LAKE CITY INTERNATIONAL'})
CREATE (oak:Airport {abbr:'OAK', id:13796, name:'METROPOLITAN OAKLAND INTERNATIONAL'})
CREATE (bur:Airport {abbr:'BUR', id:10800, name:'BOB HOPE'})
CREATE (f2:Flight {flight_num:6147, day:2, month:1, weekday:6, year:2016})
CREATE (f9:Flight {flight_num:6147, day:9, month:1, weekday:6, year:2016})
CREATE (f16:Flight {flight_num:6147, day:16, month:1, weekday:6, year:2016})
CREATE (f23:Flight {flight_num:6147, day:23, month:1, weekday:6, year:2016})
CREATE (f30:Flight {flight_num:6147, day:30, month:1, weekday:6, year:2016})
CREATE (f2)-[:DESTINATION {arr_delay:-13, taxi_time:9}]->(oak)
CREATE (f9)-[:DESTINATION {arr_delay:-8, taxi_time:4}]->(bur)
CREATE (f16)-[:DESTINATION {arr_delay:-30, taxi_time:4}]->(slc)
CREATE (f23)-[:DESTINATION {arr_delay:-21, taxi_time:3}]->(slc)
CREATE (f30)-[:DESTINATION]->(slc)
Using Manual Index on Node Properties
In order to create manual index on a node property, you call apoc.index.addNode
with the node, providing the properties to be indexed.
MATCH (a:Airport)
CALL apoc.index.addNode(a,['name'])
RETURN count(*)
The statement will create the node index with the same name as the Label name(s) of the node in this case Airport
and add the node by their properties to the index.
Once this has been added check if the node index exists using apoc.index.list
.
CALL apoc.index.list()
Usually apoc.index.addNode
would be used as part of node-creation, e.g. during LOAD CSV.
There is also apoc.index.addNodes
for adding a list of multiple nodes at once.
Once the node index is created we can start using it.
Here are some examples:
The apoc.index.nodes
finds nodes in a manual index using the given lucene query.
Note
|
That makes only sense if you combine multiple properties in one lookup or use case insensitive or fuzzy matching full-text queries. In all other cases the built in schema indexes should be used. |
CALL apoc.index.nodes('Airport','name:inter*') YIELD node AS airport, weight
RETURN airport.name, weight
LIMIT 10
Note
|
Apoc index queries not only return nodes and relationships but also a weight, which is the score returned from the underlying Lucene index. The results are also sorted by that score. That’s especially helpful for partial and fuzzy text searches. |
To remove the node index Airport
created, use:
CALL apoc.index.remove('Airport')
Using Manual Index on Relationship Properties
The procedure apoc.index.addRelationship
is used to create a manual index on relationship properties.
As there are no schema indexes for relationships, these manual indexes can be quite useful.
MATCH (:Flight)-[r:DESTINATION]->(:Airport)
CALL apoc.index.addRelationship(r,['taxi_time'])
RETURN count(*)
The statement will create the relationship index with the same name as relationship-type, in this case DESTINATION
and add the relationship by its properties to the index.
Using apoc.index.relationships
, we can find the relationship of type DESTINATION
with the property taxi_time
of 11 minutes.
We can chose to also return the start and end-node.
CALL apoc.index.relationships('DESTINATION','taxi_time:11') YIELD rel, start AS flight, end AS airport
RETURN flight_num.flight_num, airport.name;
Note
|
Manual relationship indexed do not only store the relationship by its properties but also the start- and end-node. |
That’s why we can use that information to subselect relationships not only by property but also by those nodes, which is quite powerful.
With apoc.index.in
we can pin the node with incoming relationships (end-node) to get the start nodes for all the DESTINATION
relationships.
For instance to find all flights arriving in 'SALT LAKE CITY INTERNATIONAL' with a taxi_time of 7 minutes we’d use:
MATCH (a:Airport {name:'SALT LAKE CITY INTERNATIONAL'})
CALL apoc.index.in(a,'DESTINATION','taxi_time:7') YIELD node AS flight
RETURN flight
The opposite is apoc.index.out
, which takes and binds end-nodes and returns start-nodes of relationships.
Really useful to quickly find a subset of relationships between nodes with many relationships (tens of thousands to millions) is apoc.index.between
.
Here you bind both the start and end-node and provide (or not) properties of the relationships.
MATCH (f:Flight {flight_num:6147})
MATCH (a:Airport {name:'SALT LAKE CITY INTERNATIONAL'})
CALL apoc.index.between(f,'DESTINATION',a,'taxi_time:7') YIELD rel, weight
RETURN *
To remove the relationship index DESTINATION
that was created, use.
CALL apoc.index.remove('DESTINATION')
Full Text Search
Indexes are used for finding nodes in the graph that further operations can then continue from. Just like in a book where you look at the index to find a section that interest you, and then start reading from there. A full text index allows you to find occurrences of individual words or phrases across all attributes.
In order to use the full text search feature, we have to first index our data by specifying all the attributes we want to index.
Here we create a full text index called “locations”
(we will use this name when searching in the index) with our data.
Optionally you can enable tracking changes to the graph on a per index level. To do so, you need to take two actions:
-
set
apoc.autoUpdate.enabled=true
in yourneo4j.conf
. With that setting aTransactionEventHandler
is registered upon startup of your graph database that reflects property changes to the respective fulltext index. -
By default index tracking is done synchronously within the same transaction. Optionally this can be done asynchronous by setting
apoc.autoUpdate.async=true
. -
indexing is started with:
CALL apoc.index.addAllNodes('locations',{
Company: ["name", "description"],
Person: ["name","address"],
Address: ["address"]})
Creating the index will take a little while since the procedure has to read through the entire database to create the index.
We can now use this index to search for nodes in the database. The most simple case would be to search across all data for a particular word.
It does not matter which property that word exists in, any node that has that word in any of its indexed properties will be found.
If you use a name in the call, all occurrences will be found (but limited to 100 results).
CALL apoc.index.search("locations", 'name')
Advanced Search
We can further restrict our search to only searching in a particular attribute.
In order to search for a Person
with an address in France, we use the following.
CALL apoc.index.search("locations", "Person.address:France")
Now we can search for nodes with a specific property value, and then explore their neighbourhoods visually.
But integrating it with an graph query is so much more powerful.
Fulltext and Graph Search
We could for instance search for addresses in the database that contain the word "Paris", and then find all companies registered at those addresses:
CALL apoc.index.search("locations", "Address.address:Paris~") YIELD node AS addr
MATCH (addr)<-[:HAS_ADDRESS]-(company:Company)
RETURN company LIMIT 50
The tilde (~) instructs the index search procedure to do a fuzzy match, allowing us to find “Paris” even if the spelling is slightly off.
We might notice that there are addresses that contain the word “Paris” that are not in Paris, France. For example there might be a Paris Street somewhere.
We can further specify that we want the text to contain both the word Paris, and the word France:
CALL apoc.index.search("locations", "+Address.address:Paris~ +France~")
YIELD node AS addr
MATCH (addr)<-[:HAS_ADDRESS]-(company:Company)
RETURN company LIMIT 50
Complex Searches
Things start to get interesting when we look at how the different entities in Paris are connected to one another. We can do that by finding all the entities with addresses in Paris, then creating all pairs of such entities and finding the shortest path between each such pair:
CALL apoc.index.search("locations", "+Address.address:Paris~ +France~") YIELD node AS addr
MATCH (addr)<-[:HAS_ADDRESS]-(company:Company)
WITH collect(company) AS companies
// create unique pairs
UNWIND companies AS x UNWIND companies AS y
WITH x, y WHERE ID(x) < ID(y)
MATCH path = shortestPath((x)-[*..10]-(y))
RETURN path
For more details on the query syntax used in the second parameter of the search
procedure,
please see this Lucene query tutorial
Utility Functions
Phonetic Text Procedures
The phonetic text (soundex) procedures allow you to compute the soundex encoding of a given string. There is also a procedure to compare how similar two strings sound under the soundex algorithm. All soundex procedures by default assume the used language is US English.
CALL apoc.text.phonetic('Hello, dear User!') YIELD value
RETURN value // will return 'H436'
CALL apoc.text.phoneticDelta('Hello Mr Rabbit', 'Hello Mr Ribbit') // will return '4' (very similar)
Extract Domain
The procedure apoc.data.domain
will take a url or email address and try to determine the domain name.
This can be useful to make easier correlations and equality tests between differently formatted email addresses, and between urls to the same domains but specifying different locations.
WITH 'foo@bar.com' AS email
CALL apoc.data.domain(email) YIELD domain
RETURN domain // will return 'bar.com'
WITH 'http://www.example.com/all-the-things' AS url
CALL apoc.data.domain(url) YIELD domain
RETURN domain // will return 'www.example.com'
TimeToLive (TTL) - Expire Nodes
Enable cleanup of expired nodes in neo4j.conf
with apoc.ttl.enabled=true
30s after startup an index is created:
CREATE INDEX ON :TTL(ttl)
At startup a statement is scheduled to run every 60s (or configure in neo4j.conf
- apoc.ttl.schedule=120
)
MATCH (t:TTL) where t.ttl < timestamp() WITH t LIMIT 1000 DETACH DELETE t
The ttl
property holds the time when the node is expired in milliseconds since epoch.
You can expire your nodes by setting the :TTL label and the ttl property:
MATCH (n:Foo) WHERE n.bar SET n:TTL, n.ttl = timestamp() + 10000;
There is also a procedure that does the same:
CALL apoc.date.expire(node,time,'time-unit');
CALL apoc.date.expire(n,100,'s');
Date and Time Conversions
(thanks @tkroman)
Conversion between formatted dates and timestamps
-
apoc.date.parseDefault('2015-03-25 03:15:59','s')
get Unix time equivalent of given date (in seconds) -
apoc.date.parse('2015/03/25 03-15-59','s','yyyy/MM/dd HH/mm/ss')
same as previous, but accepts custom datetime format -
apoc.date.formatDefault(12345,'s')
get string representation of date corresponding to given Unix time (in seconds) in UTC time zone -
apoc.date.format(12345,'s', 'yyyy/MM/dd HH/mm/ss')
the same as previous, but accepts custom datetime format -
apoc.date.formatTimeZone(12345,'s', 'yyyy/MM/dd HH/mm/ss', 'ABC')
the same as previous, but accepts custom time zone -
possible unit values:
ms,s,m,h,d
and their long forms. -
possible time zone values: Either an abbreviation such as
PST
, a full name such asAmerica/Los_Angeles
, or a custom ID such asGMT-8:00
. Full names are recommended.
Reading separate datetime fields:
Splits date (optionally, using given custom format) into fields returning a map from field name to its value.
CALL apoc.date.fields('2015-03-25 03:15:59')
CALL apoc.date.fieldsFormatted('2015-01-02 03:04:05 EET', 'yyyy-MM-dd HH:mm:ss zzz')
Following fields are supported:
Result field | Represents |
---|---|
'years' |
year |
'months' |
month of year |
'days' |
day of month |
'hours' |
hour of day |
'minutes' |
minute of hour |
'seconds' |
second of minute |
'zone' |
Examples
CALL apoc.date.fieldsDefault('2015-03-25 03:15:59')
{ 'Months': 1, 'Days': 2, 'Hours': 3, 'Minutes': 4, 'Seconds': 5, 'Years': 2015 }
CALL apoc.date.fields('2015-01-02 03:04:05 EET', 'yyyy-MM-dd HH:mm:ss zzz')
{ 'ZoneId': 'Europe/Bucharest', 'Months': 1, 'Days': 2, 'Hours': 3, 'Minutes': 4, 'Seconds': 5, 'Years': 2015 }
CALL apoc.date.fields('2015/01/02_EET', 'yyyy/MM/dd_z')
{ 'Years': 2015, 'ZoneId': 'Europe/Bucharest', 'Months': 1, 'Days': 2 }
Notes on formats:
-
the default format is
yyyy-MM-dd HH:mm:ss
-
if the format pattern doesn’t specify timezone, formatter considers dates to belong to the UTC timezone
-
if the timezone pattern is specified, the timezone is extracted from the date string, otherwise an error will be reported
-
the
to/fromSeconds
timestamp values are in POSIX (Unix time) system, i.e. timestamps represent the number of seconds elapsed since 00:00:00 UTC, Thursday, 1 January 1970 -
the full list of supported formats is described in SimpleDateFormat JavaDoc
Number Format Conversions
Conversion between formatted decimals
-
apoc.number.format(number) yield value
format a long or double using the default system pattern and language to produce a string -
apoc.number.format.pattern(number, pattern) yield value
format a long or double using a pattern and the default system language to produce a string -
apoc.number.format.lang(number, lang) yield value
format a long or double using the default system pattern pattern and a language to produce a string -
apoc.number.format.pattern.lang(number, pattern, lang) yield value
format a long or double using a pattern and a language to produce a string -
apoc.number.parseInt(text) yield value
parse a text using the default system pattern and language to produce a long -
apoc.number.parseInt.pattern(text, pattern) yield value
parse a text using a pattern and the default system language to produce a long -
apoc.number.parseInt.lang(text, lang) yield value
parse a text using the default system pattern and a language to produce a long -
apoc.number.parseInt.pattern.lang(text, pattern, lang) yield value
parse a text using a pattern and a language to produce a long -
apoc.number.parseFloat(text) yield value
parse a text using the default system pattern and language to produce a double -
apoc.number.parseFloat.pattern(text, pattern) yield value
parse a text using a pattern and the default system language to produce a double -
apoc.number.parseFloat.lang(text, lang) yield value
parse a text using the default system pattern and a language to produce a double -
apoc.number.parseFloat.pattern.lang(text, pattern, lang) yield value
parse a text using a pattern and a language to produce a double -
The full list of supported values for
pattern
andlang
params is described in DecimalFormat JavaDoc
Examples
call apoc.number.format(12345.67) yield value return value ╒═════════╕ │value │ ╞═════════╡ │12,345.67│ └─────────┘
call apoc.number.format.pattern.lang(12345, '#,##0.00;(#,##0.00)', 'it') yield value return value ╒═════════╕ │value │ ╞═════════╡ │12.345,00│ └─────────
call apoc.number.format.pattern.lang(12345.67, '#,##0.00;(#,##0.00)', 'it') yield value return value ╒═════════╕ │value │ ╞═════════╡ │12.345,67│ └─────────┘
call apoc.number.parseInt.pattern.lang('12.345', '#,##0.00;(#,##0.00)', 'it') yield value return value ╒═════╕ │value│ ╞═════╡ │12345│ └─────┘
call apoc.number.parseFloat.pattern.lang('12.345,67', '#,##0.00;(#,##0.00)', 'it') yield value return value ╒════════╕ │value │ ╞════════╡ │12345.67│ └────────┘
call apoc.number.format('aaa') yield value Failed to invoke procedure `apoc.number.format`: Caused by: java.lang.IllegalArgumentException: Number parameter must be long or double.
call apoc.number.format.lang(12345, 'apoc') Failed to invoke procedure `apoc.number.format.lang`: Caused by: java.lang.IllegalArgumentException: Unrecognized language value: 'apoc' isn't a valid ISO language
call apoc.number.parseInt('aaa') Failed to invoke procedure `apoc.number.parseAsLong`: Caused by: java.text.ParseException: Unparseable number: "aaa"
Graph Algorithms
Algorithm Procedures
Community Detection via Label Propagation
APOC includes a simple procedure for label propagation. It may be used to detect communities or solve other graph partitioning problems. The following example shows how it may be used.
The example call with scan all nodes 25 times. During a scan the procedure will look at all outgoing relationships of type :X for each node n. For each of these relationships, it will compute a weight and use that as a vote for the other node’s 'partition' property value. Finally, n.partition is set to the property value that acquired the most votes.
Weights are computed by multiplying the relationship weight with the weight of the other nodes. Both weights are taken from the 'weight' property; if no such property is found, the weight is assumed to be 1.0. Similarly, if no 'weight' property key was specified, all weights are assumed to be 1.0.
CALL apoc.algo.community(25,null,'partition','X','OUTGOING','weight',10000)
The second argument is a list of label names and may be used to restrict which nodes are scanned.
Expand paths
expand from start node following the given relationships from min to max-level adhering to the label filters
Usage
CALL apoc.path.expand(startNode <id>|Node, relationshipFilter, labelFilter, minLevel, maxLevel )
CALL apoc.path.expand(startNode <id>|Node|list, 'TYPE|TYPE_OUT>|<TYPE_IN', '+YesLabel|-NoLabel', minLevel, maxLevel ) yield path
Relationship Filter
Syntax: [<]RELATIONSHIP_TYPE1[>]|[<]RELATIONSHIP_TYPE2[>]|…
input | type | direction |
---|---|---|
|
|
Table of Contents
OUTGOING |
|
|
Table of Contents
INCOMING |
|
|
Table of Contents
BOTH |
Label Filter
Syntax: [+-/]LABEL1|LABEL2|…
input | label | result |
---|---|---|
|
|
Table of Contents
include label (whitelist) |
|
|
Table of Contents
exclude label (blacklist) |
|
|
Table of Contents
stop traversal after reaching a friend (but include him) |
Examples
call apoc.path.expand(1,"ACTED_IN>|PRODUCED<|FOLLOWS<","+Movie|Person",0,3)
call apoc.path.expand(1,"ACTED_IN>|PRODUCED<|FOLLOWS<","-BigBrother",0,3)
call apoc.path.expand(1,"ACTED_IN>|PRODUCED<|FOLLOWS<","",0,3)
// combined with cypher:
match (tom:Person {name :"Tom Hanks"})
call apoc.path.expand(tom,"ACTED_IN>|PRODUCED<|FOLLOWS<","+Movie|Person",0,3) yield path as pp
return pp;
// or
match (p:Person) with p limit 3
call apoc.path.expand(p,"ACTED_IN>|PRODUCED<|FOLLOWS<","+Movie|Person",1,2) yield path as pp
return p, pp
Expand with Config
apoc.path.expandConfig(startNode <id>Node/list, {config}) yield path expands from start nodes using the given configuration and yields the resulting paths
Takes an additional config to provide configuration options:
{minLevel: -1|number, maxLevel: -1|number, relationshipFilter: '[<]RELATIONSHIP_TYPE1[>]|[<]RELATIONSHIP_TYPE2[>]|...', labelFilter: '[+-]LABEL1|LABEL2|...', uniqueness: RELATIONSHIP_PATH|NONE|NODE_GLOBAL|NODE_LEVEL|NODE_PATH|NODE_RECENT|RELATIONSHIP_GLOBAL|RELATIONSHIP_LEVEL|RELATIONSHIP_RECENT, bfs: true|false}
So you can turn this cypher query:
MATCH (user:User) WHERE user.id = 460
MATCH (user)-[:RATED]->(movie)<-[:RATED]-(collab)-[:RATED]->(reco)
RETURN count(*);
into this procedure call, with changed semantics for uniqueness and bfs (which is Cypher’s expand mode)
MATCH (user:User) WHERE user.id = 460
CALL apoc.path.expandConfig(user,{relationshipFilter:"RATED",minLevel:3,maxLevel:3,bfs:false,uniqueness:"NONE"}) YIELD path
RETURN count(*);
Centrality Algorithms
Setup
Let’s create some test data to run the Centrality algorithms on.
// create 100 nodes
FOREACH (id IN range(0,1000) | CREATE (:Node {id:id}))
// over the cross product (1M) create 100.000 relationships
MATCH (n1:Node),(n2:Node) WITH n1,n2 LIMIT 1000000 WHERE rand() < 0.1
CREATE (n1)-[:TYPE]->(n2)
Closeness Centrality Procedure
Centrality is an indicator of a node’s influence in a graph. In graphs there is a natural distance metric between pairs of nodes, defined by the length of their shortest paths. For both algorithms below we can measure based upon the direction of the relationship, whereby the 3rd argument represents the direction and can be of value BOTH, INCOMING, OUTGOING.
Closeness Centrality defines the farness of a node as the sum of its distances from all other nodes, and its closeness as the reciprocal of farness.
The more central a node is the lower its total distance from all other nodes.
Complexity: This procedure uses a BFS shortest path algorithm. With BFS the complexes becomes O(n * m)
Caution: Due to the complexity of this algorithm it is recommended to run it on only the nodes you are interested in.
MATCH (node:Node)
WHERE node.id %2 = 0
WITH collect(node) AS nodes
CALL apoc.algo.closeness(['TYPE'],nodes,'INCOMING') YIELD node, score
RETURN node, score
ORDER BY score DESC
Betweenness Centrality Procedure
The procedure will compute betweenness centrality as defined by Linton C. Freeman (1977) using the algorithm by Ulrik Brandes (2001). Centrality is an indicator of a node’s influence in a graph.
Betweenness Centrality is equal to the number of shortest paths from all nodes to all others that pass through that node.
High centrality suggests a large influence on the transfer of items through the graph.
Centrality is applicable to numerous domains, including: social networks, biology, transport and scientific cooperation.
Complexity: This procedure uses a BFS shortest path algorithm. With BFS the complexes becomes O(n * m) Caution: Due to the complexity of this algorithm it is recommended to run it on only the nodes you are interested in.
MATCH (node:Node)
WHERE node.id %2 = 0
WITH collect(node) AS nodes
CALL apoc.algo.betweenness(['TYPE'],nodes,'BOTH') YIELD node, score
RETURN node, score
ORDER BY score DESC
PageRank Algorithm
Setup
Let’s create some test data to run the PageRank algorithm on.
// create 100 nodes
FOREACH (id IN range(0,1000) | CREATE (:Node {id:id}))
// over the cross product (1M) create 100.000 relationships
MATCH (n1:Node),(n2:Node) WITH n1,n2 LIMIT 1000000 WHERE rand() < 0.1
CREATE (n1)-[:TYPE_1]->(n2)
PageRank Procedure
PageRank is an algorithm used by Google Search to rank websites in their search engine results.
It is a way of measuring the importance of nodes in a graph.
PageRank counts the number and quality of relationships to a node to approximate the importance of that node.
PageRank assumes that more important nodes likely have more relationships.
Caution: nodes
specifies the nodes for which a PageRank score will be projected, but the procedure will always compute the PageRank algorithm on the entire graph. At present, there is no way to filter/reduce the number of elements that PageRank computes over.
A future version of this procedure will provide the option of computing PageRank on a subset of the graph.
MATCH (node:Node)
WHERE node.id %2 = 0
WITH collect(node) AS nodes
// compute over relationships of all types
CALL apoc.algo.pageRank(nodes) YIELD node, score
RETURN node, score
ORDER BY score DESC
MATCH (node:Node)
WHERE node.id %2 = 0
WITH collect(node) AS nodes
// only compute over relationships of types TYPE_1 or TYPE_2
CALL apoc.algo.pageRankWithConfig(nodes,{types:'TYPE_1|TYPE_2'}) YIELD node, score
RETURN node, score
ORDER BY score DESC
MATCH (node:Node)
WHERE node.id %2 = 0
WITH collect(node) AS nodes
// peroform 10 page rank iterations, computing only over relationships of type TYPE_1
CALL apoc.algo.pageRankWithConfig(nodes,{iterations:10,types:'TYPE_1'}) YIELD node, score
RETURN node, score
ORDER BY score DESC
Spatial
Spatial Functions
The spatial procedures are intended to enable geographic capabilities on your data.
geocode
The first procedure geocode which will convert a textual address into a location containing latitude, longitude and description. Despite being only a single function, together with the built-in functions point and distance we can achieve quite powerful results.
First, how can we use the procedure:
CALL apoc.spatial.geocodeOnce('21 rue Paul Bellamy 44000 NANTES FRANCE') YIELD location
RETURN location.latitude, location.longitude // will return 47.2221667, -1.5566624
There are two forms of the procedure:
-
geocodeOnce(address) returns zero or one result
-
geocode(address,maxResults) returns zero, one or more up to maxResults
This is because the backing geocoding service (OSM, Google, OpenCage or other) might return multiple results for the same query. GeocodeOnce() is designed to return the first, or highest ranking result.
Configuring Geocode
There are a few options that can be set in the neo4j.conf file to control the service:
-
apoc.spatial.geocode.provider=osm (osm, google, opencage, etc.)
-
apoc.spatial.geocode.osm.throttle=5000 (ms to delay between queries to not overload OSM servers)
-
apoc.spatial.geocode.google.throttle=1 (ms to delay between queries to not overload Google servers)
-
apoc.spatial.geocode.google.key=xxxx (API key for google geocode access)
-
apoc.spatial.geocode.google.client=xxxx (client code for google geocode access)
-
apoc.spatial.geocode.google.signature=xxxx (client signature for google geocode access)
For google, you should use either a key or a combination of client and signature. Read more about this on the google page for geocode access at https://developers.google.com/maps/documentation/geocoding/get-api-key#key
Configuring Custom Geocode Provider
For any provider that is not 'osm' or 'google' you get a configurable supplier that requires two additional settings, 'url' and 'key'. The 'url' must contain the two words 'PLACE' and 'KEY'. The 'KEY' will be replaced with the key you get from the provider when you register for the service. The 'PLACE' will be replaced with the address to geocode when the procedure is called.
For example, to get the service working with OpenCage, perform the following steps:
-
Register your own application key at https://geocoder.opencagedata.com/
-
Once you have a key, add the following three lines to neo4j.conf
apoc.spatial.geocode.provider=opencage apoc.spatial.geocode.opencage.key=XXXXXXXXXXXXXXXXXXXXXXXXXXXXXX apoc.spatial.geocode.opencage.url=http://api.opencagedata.com/geocode/v1/json?q=PLACE&key=KEY
-
make sure that the 'XXXXXXX' part above is replaced with your actual key
-
Restart the Neo4j server and then test the geocode procedures to see that they work
-
If you are unsure if the provider is correctly configured try verify with:
CALL apoc.spatial.showConfig()
Using Geocode within a bigger Cypher query
A more complex, or useful, example which geocodes addresses found in properties of nodes:
MATCH (a:Place)
WHERE exists(a.address)
CALL apoc.spatial.geocodeOnce(a.address) YIELD location
RETURN location.latitude AS latitude, location.longitude AS longitude, location.description AS description
Calculating distance between locations
If we wish to calculate the distance between addresses, we need to use the point() function to convert latitude and longitude to Cyper Point types, and then use the distance() function to calculate the distance:
WITH point({latitude: 48.8582532, longitude: 2.294287}) AS eiffel
MATCH (a:Place)
WHERE exists(a.address)
CALL apoc.spatial.geocodeOnce(a.address) YIELD location
WITH location, distance(point(location), eiffel) AS distance
WHERE distance < 5000
RETURN location.description AS description, distance
ORDER BY distance
LIMIT 100
sortPathsByDistance
The second procedure enables you to sort a given collection of paths by the sum of their distance based on lat/long properties on the nodes.
Sample data :
CREATE (bruges:City {name:"bruges", latitude: 51.2605829, longitude: 3.0817189})
CREATE (brussels:City {name:"brussels", latitude: 50.854954, longitude: 4.3051786})
CREATE (paris:City {name:"paris", latitude: 48.8588376, longitude: 2.2773455})
CREATE (dresden:City {name:"dresden", latitude: 51.0767496, longitude: 13.6321595})
MERGE (bruges)-[:NEXT]->(brussels)
MERGE (brussels)-[:NEXT]->(dresden)
MERGE (brussels)-[:NEXT]->(paris)
MERGE (bruges)-[:NEXT]->(paris)
MERGE (paris)-[:NEXT]->(dresden)
Finding paths and sort them by distance
MATCH (a:City {name:'bruges'}), (b:City {name:'dresden'})
MATCH p=(a)-[*]->(b)
WITH collect(p) as paths
CALL apoc.spatial.sortPathsByDistance(paths) YIELD path, distance
RETURN path, distance
Graph Refactoring
In order not to have to repeatedly geocode the same thing in multiple queries, especially if the database will be used by many people, it might be a good idea to persist the results in the database so that subsequent calls can use the saved results.
Geocode and persist the result
MATCH (a:Place)
WHERE exists(a.address) AND NOT exists(a.latitude)
WITH a LIMIT 1000
CALL apoc.spatial.geocodeOnce(a.address) YIELD location
SET a.latitude = location.latitude
SET a.longitude = location.longitude
Note that the above command only geocodes the first 1000 ‘Place’ nodes that have not already been geocoded. This query can be run multiple times until all places are geocoded. Why would we want to do this? Two good reasons:
-
The geocoding service is a public service that can throttle or blacklist sites that hit the service too heavily, so controlling how much we do is useful.
-
The transaction is updating the database, and it is wise not to update the database with too many things in the same transaction, to avoid using up too much memory. This trick will keep the memory usage very low.
Now make use of the results in distance queries
WITH point({latitude: 48.8582532, longitude: 2.294287}) AS eiffel
MATCH (a:Place)
WHERE exists(a.latitude) AND exists(a.longitude)
WITH a, distance(point(a), eiffel) AS distance
WHERE distance < 5000
RETURN a.name, distance
ORDER BY distance
LIMIT 100
Combined Space and Time search
Combining spatial and date-time procedures can allow for more complex queries:
CALL apoc.date.parse('2016-06-01 00:00:00','h') YIELD value AS due_date
WITH due_date,
point({latitude: 48.8582532, longitude: 2.294287}) AS eiffel
MATCH (e:Event)
WHERE exists(e.address) AND exists(e.datetime)
CALL apoc.spatial.geocodeOnce(e.address) YIELD location
CALL apoc.date.parse(e.datetime,'h') YIELD value AS hours
WITH e, location,
distance(point(location), eiffel) AS distance,
(due_date - hours)/24.0 AS days_before_due
WHERE distance < 5000 AND days_before_due < 14 AND hours < due_date
RETURN e.name AS event, e.datetime AS date,
location.description AS description, distance
ORDER BY distance
Data Integration
Load JSON
Load JSON
Web APIs are a huge opportunity to access and integrate data from any sources with your graph. Most of them provide the data as JSON.
With apoc.load.json
you can retrieve data from URLs and turn it into map value(s) for Cypher to consume.
Cypher is pretty good at deconstructing nested documents with dot syntax, slices, UNWIND
etc. so it is easy to turn nested data into graphs.
Load JSON StackOverflow Example
There have been articles before about loading JSON from Web-APIs like StackOverflow.
With apoc.load.json
it’s now very easy to load JSON data from any file or URL.
If the result is a JSON object is returned as a singular map. Otherwise if it was an array is turned into a stream of maps.
The URL for retrieving the last questions and answers of the neo4j tag is this:
Now it can be used from within Cypher directly, let’s first introspect the data that is returned.
WITH "https://api.stackexchange.com/2.2/questions?pagesize=100&order=desc&sort=creation&tagged=neo4j&site=stackoverflow&filter=!5-i6Zw8Y)4W7vpy91PMYsKM-k9yzEsSC1_Uxlf" AS url
CALL apoc.load.json(url) YIELD value
UNWIND value.items AS item
RETURN item.title, item.owner, item.creation_date, keys(item)
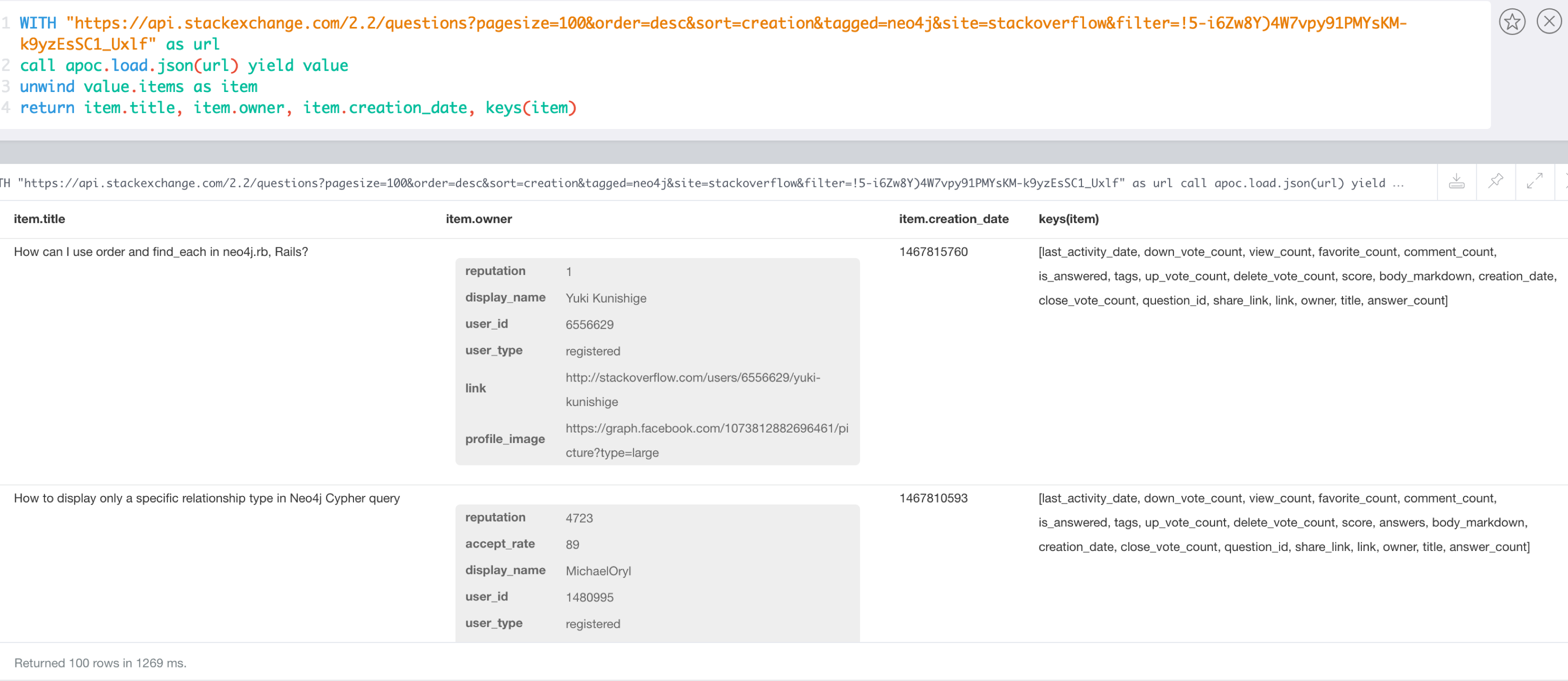
Combined with the cypher query from the original blog post it’s easy to create the full Neo4j graph of those entities.
WITH "https://api.stackexchange.com/2.2/questions?pagesize=100&order=desc&sort=creation&tagged=neo4j&site=stackoverflow&filter=!5-i6Zw8Y)4W7vpy91PMYsKM-k9yzEsSC1_Uxlf" AS url
CALL apoc.load.json(url) YIELD value
UNWIND value.items AS q
MERGE (question:Question {id:q.question_id}) ON CREATE
SET question.title = q.title, question.share_link = q.share_link, question.favorite_count = q.favorite_count
MERGE (owner:User {id:q.owner.user_id}) ON CREATE SET owner.display_name = q.owner.display_name
MERGE (owner)-[:ASKED]->(question)
FOREACH (tagName IN q.tags | MERGE (tag:Tag {name:tagName}) MERGE (question)-[:TAGGED]->(tag))
FOREACH (a IN q.answers |
MERGE (question)<-[:ANSWERS]-(answer:Answer {id:a.answer_id})
MERGE (answerer:User {id:a.owner.user_id}) ON CREATE SET answerer.display_name = a.owner.display_name
MERGE (answer)<-[:PROVIDED]-(answerer)
)
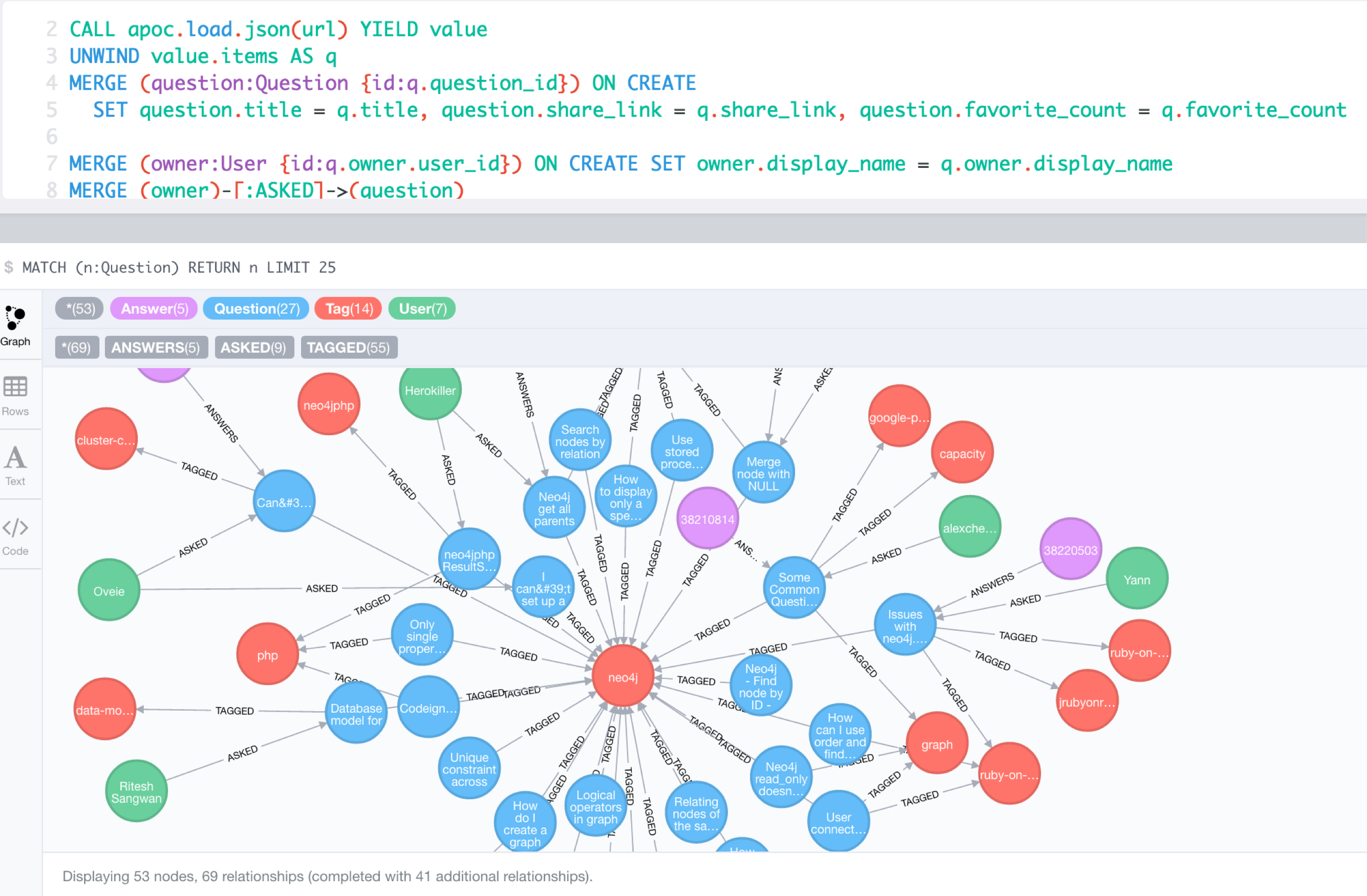
Load JSON from Twitter (with additional parameters)
With apoc.load.jsonParams
you can send additional headers or payload with your JSON GET request, e.g. for the Twitter API:
Configure Bearer and Twitter Search Url token in neo4j.conf
apoc.static.twitter.bearer=XXXX apoc.static.twitter.url=https://api.twitter.com/1.1/search/tweets.json?count=100&result_type=recent&lang=en&q=
CALL apoc.static.getAll("twitter") yield value AS twitter
CALL apoc.load.jsonParams(twitter.url + "oscon+OR+neo4j+OR+%23oscon+OR+%40neo4j",{Authorization:"Bearer "+twitter.bearer},null) yield value
UNWIND value.statuses as status
WITH status, status.user as u, status.entities as e
RETURN status.id, status.text, u.screen_name, [t IN e.hashtags | t.text] as tags, e.symbols, [m IN e.user_mentions | m.screen_name] as mentions, [u IN e.urls | u.expanded_url] as urls
GeoCoding Example
Example for reverse geocoding and determining the route from one to another location.
WITH
"21 rue Paul Bellamy 44000 NANTES FRANCE" AS fromAddr,
"125 rue du docteur guichard 49000 ANGERS FRANCE" AS toAddr
call apoc.load.json("http://www.yournavigation.org/transport.php?url=http://nominatim.openstreetmap.org/search&format=json&q=" + replace(fromAddr, ' ', '%20')) YIELD value AS from
WITH from, toAddr LIMIT 1
call apoc.load.json("http://www.yournavigation.org/transport.php?url=http://nominatim.openstreetmap.org/search&format=json&q=" + replace(toAddr, ' ', '%20')) YIELD value AS to
CALL apoc.load.json("https://router.project-osrm.org/viaroute?instructions=true&alt=true&z=17&loc=" + from.lat + "," + from.lon + "&loc=" + to.lat + "," + to.lon ) YIELD value AS doc
UNWIND doc.route_instructions as instruction
RETURN instruction
Load JDBC
Overview: Database Integration
Data Integration is an important topic. Reading data from relational databases to create and augment data models is a very helpful exercise.
With apoc.load.jdbc
you can access any database that provides a JDBC driver, and execute queries whose results are turned into streams of rows.
Those rows can then be used to update or create graph structures.
|
load from relational database, either a full table or a sql statement |
|
load from relational database, either a full table or a sql statement |
|
register JDBC driver of source database |
To simplify the JDBC URL syntax and protect credentials, you can configure aliases in conf/neo4j.conf
:
apoc.jdbc.myDB.url=jdbc:derby:derbyDB
CALL apoc.load.jdbc('jdbc:derby:derbyDB','PERSON') becomes CALL apoc.load.jdbc('myDB','PERSON')
The 3rd value in the apoc.jdbc.<alias>.url=
effectively defines an alias to be used in apoc.load.jdbc('<alias>',….
MySQL Example
Northwind is a common example set for relational databases, which is also covered in our import guides, e.g. :play northwind graph in the Neo4j browser.
MySQL Northwind Data
select count(*) from products; +----------+ | count(*) | +----------+ | 77 | +----------+ 1 row in set (0,00 sec)
describe products; +-----------------+---------------+------+-----+---------+----------------+ | Field | Type | Null | Key | Default | Extra | +-----------------+---------------+------+-----+---------+----------------+ | ProductID | int(11) | NO | PRI | NULL | auto_increment | | ProductName | varchar(40) | NO | MUL | NULL | | | SupplierID | int(11) | YES | MUL | NULL | | | CategoryID | int(11) | YES | MUL | NULL | | | QuantityPerUnit | varchar(20) | YES | | NULL | | | UnitPrice | decimal(10,4) | YES | | 0.0000 | | | UnitsInStock | smallint(2) | YES | | 0 | | | UnitsOnOrder | smallint(2) | YES | | 0 | | | ReorderLevel | smallint(2) | YES | | 0 | | | Discontinued | bit(1) | NO | | b'0' | | +-----------------+---------------+------+-----+---------+----------------+ 10 rows in set (0,00 sec)
Load JDBC Examples
cypher CALL apoc.load.driver("com.mysql.jdbc.Driver");
with "jdbc:mysql://localhost:3306/northwind?user=root" as url
cypher CALL apoc.load.jdbc(url,"products") YIELD row
RETURN count(*);
+----------+ | count(*) | +----------+ | 77 | +----------+ 1 row 23 ms
with "jdbc:mysql://localhost:3306/northwind?user=root" as url
cypher CALL apoc.load.jdbc(url,"products") YIELD row
RETURN row limit 1;
+--------------------------------------------------------------------------------+ | row | +--------------------------------------------------------------------------------+ | {UnitPrice -> 18.0000, UnitsOnOrder -> 0, CategoryID -> 1, UnitsInStock -> 39} | +--------------------------------------------------------------------------------+ 1 row 10 ms
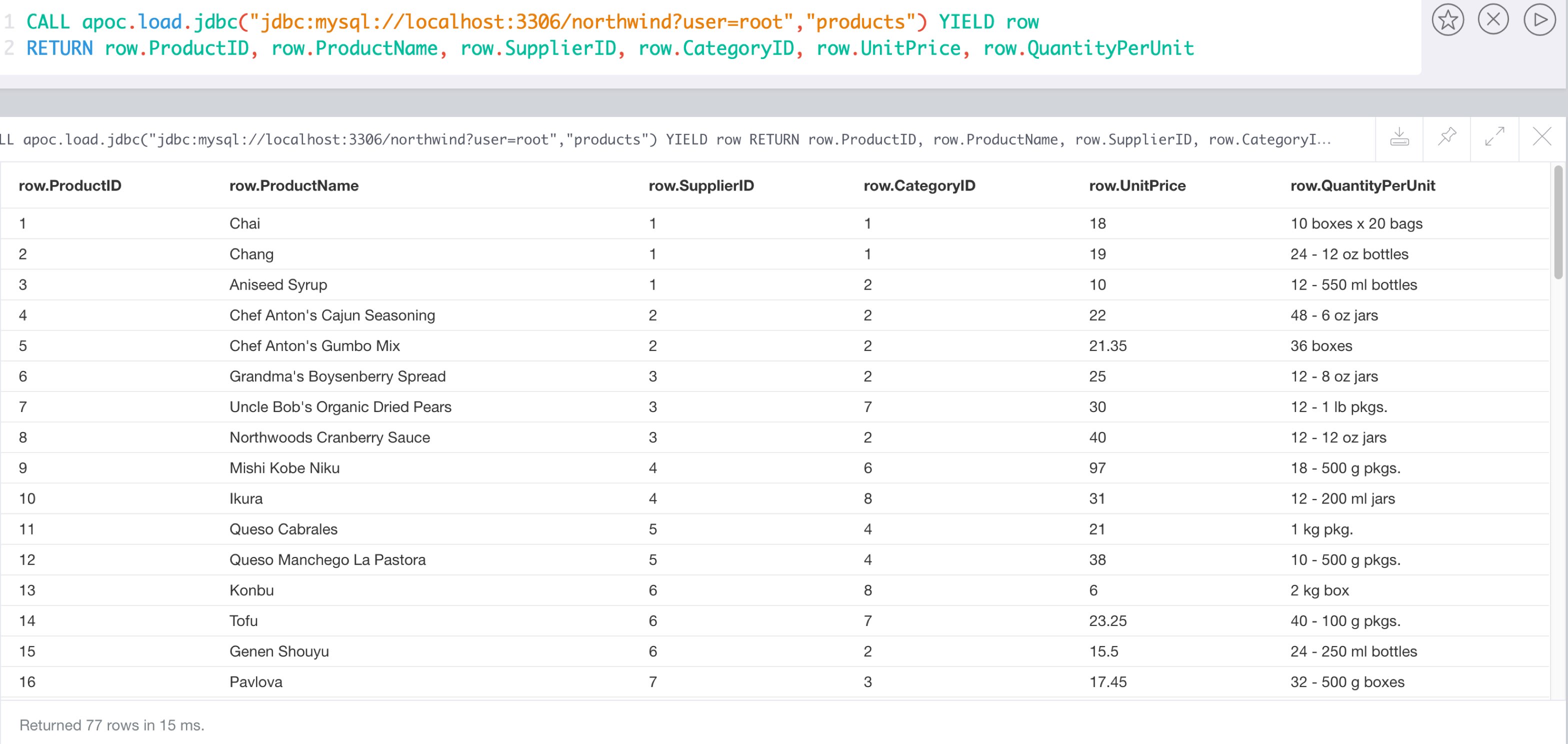
Load data in transactional batches
You can load data from jdbc and create/update the graph using the query results in batches (and in parallel).
CALL apoc.periodic.iterate('
call apoc.load.jdbc("jdbc:mysql://localhost:3306/northwind?user=root","company")',
'CREATE (p:Person) SET p += value', {batchSize:10000, parallel:true})
RETURN batches, total
Cassandra Example
Setup Song database as initial dataset
curl -OL https://raw.githubusercontent.com/neo4j-contrib/neo4j-cassandra-connector/master/db_gen/playlist.cql curl -OL https://raw.githubusercontent.com/neo4j-contrib/neo4j-cassandra-connector/master/db_gen/artists.csv curl -OL https://raw.githubusercontent.com/neo4j-contrib/neo4j-cassandra-connector/master/db_gen/songs.csv $CASSANDRA_HOME/bin/cassandra $CASSANDRA_HOME/bin/cqlsh -f playlist.cql
Download the Cassandra JDBC Wrapper, and put it into your $NEO4J_HOME/plugins
directory.
Add this config option to $NEO4J_HOME/conf/neo4j.conf
to make it easier to interact with the cassandra instance.
apoc.jdbc.cassandra_songs.url=jdbc:cassandra://localhost:9042/playlist
Restart the server.
Now you can inspect the data in Cassandra with.
CALL apoc.load.jdbc('cassandra_songs','artists_by_first_letter') yield row
RETURN count(*);
╒════════╕ │count(*)│ ╞════════╡ │3605 │ └────────┘
CALL apoc.load.jdbc('cassandra_songs','artists_by_first_letter') yield row
RETURN row LIMIT 5;
CALL apoc.load.jdbc('cassandra_songs','artists_by_first_letter') yield row
RETURN row.first_letter, row.artist LIMIT 5;
╒════════════════╤═══════════════════════════════╕ │row.first_letter│row.artist │ ╞════════════════╪═══════════════════════════════╡ │C │C.W. Stoneking │ ├────────────────┼───────────────────────────────┤ │C │CH2K │ ├────────────────┼───────────────────────────────┤ │C │CHARLIE HUNTER WITH LEON PARKER│ ├────────────────┼───────────────────────────────┤ │C │Calvin Harris │ ├────────────────┼───────────────────────────────┤ │C │Camané │ └────────────────┴───────────────────────────────┘
Let’s create some graph data, we have a look at the track_by_artist table, which contains about 60k records.
CALL apoc.load.jdbc('cassandra_songs','track_by_artist') yield row RETURN count(*);
CALL apoc.load.jdbc('cassandra_songs','track_by_artist') yield row
RETURN row LIMIT 5;
CALL apoc.load.jdbc('cassandra_songs','track_by_artist') yield row
RETURN row.track_id, row.track_length_in_seconds, row.track, row.music_file, row.genre, row.artist, row.starred LIMIT 2;
╒════════════════════════════════════╤══════╤════════════════╤══════════════════╤═════════╤════════════════════════════╤═══════════╕ │row.track_id │length│row.track │row.music_file │row.genre│row.artist │row.starred│ ╞════════════════════════════════════╪══════╪════════════════╪══════════════════╪═════════╪════════════════════════════╪═══════════╡ │c0693b1e-0eaa-4e81-b23f-b083db303842│219 │1913 Massacre │TRYKHMD128F934154C│folk │Woody Guthrie & Jack Elliott│false │ ├────────────────────────────────────┼──────┼────────────────┼──────────────────┼─────────┼────────────────────────────┼───────────┤ │7d114937-0bc7-41c7-8e0c-94b5654ac77f│178 │Alabammy Bound │TRMQLPV128F934152B│folk │Woody Guthrie & Jack Elliott│false │ └────────────────────────────────────┴──────┴────────────────┴──────────────────┴─────────┴────────────────────────────┴───────────┘
Let’s create some indexes and constraints, note that other indexes and constraints will be dropped by this.
CALL apoc.schema.assert(
{Track:['title','length']},
{Artist:['name'],Track:['id'],Genre:['name']});
╒════════════╤═══════╤══════╤═══════╕ │label │key │unique│action │ ╞════════════╪═══════╪══════╪═══════╡ │Track │title │false │CREATED│ ├────────────┼───────┼──────┼───────┤ │Track │length │false │CREATED│ ├────────────┼───────┼──────┼───────┤ │Artist │name │true │CREATED│ ├────────────┼───────┼──────┼───────┤ │Genre │name │true │CREATED│ ├────────────┼───────┼──────┼───────┤ │Track │id │true │CREATED│ └────────────┴───────┴──────┴───────┘
CALL apoc.load.jdbc('cassandra_songs','track_by_artist') yield row
MERGE (a:Artist {name:row.artist})
MERGE (g:Genre {name:row.genre})
CREATE (t:Track {id:toString(row.track_id), title:row.track, length:row.track_length_in_seconds})
CREATE (a)-[:PERFORMED]->(t)
CREATE (t)-[:GENRE]->(g);
Added 63213 labels, created 63213 nodes, set 182413 properties, created 119200 relationships, statement executed in 40076 ms.
LOAD JDBC - Resources
To use other JDBC drivers use these download links and JDBC URL.
Put the JDBC driver into the $NEO4J_HOME/plugins
directory and configure the JDBC-URL in $NEO4J_HOME/conf/neo4j.conf
with apoc.jdbc.<alias>.url=<jdbc-url>
Database | JDBC-URL | Driver Source |
---|---|---|
Table of Contents
MySQL |
|
Table of Contents
|
Table of Contents
Postgres |
|
Table of Contents
|
Table of Contents
Oracle |
|
Table of Contents
|
Table of Contents
MS SQLServer |
|
Table of Contents
|
Table of Contents
IBM DB2 |
|
Table of Contents
|
Table of Contents
Derby |
|
Table of Contents
Included in JDK6-8 |
Table of Contents
Cassandra |
|
Table of Contents
|
There are a number of blog posts / examples that details usage of apoc.load.jdbc
Streaming Data to Gephi
|
streams provided data to Gephi |
Notes
Gephi has a streaming plugin, that can provide and accept JSON-graph-data in a streaming fashion.
Make sure to install the plugin firsrt and activate it for your workspace (there is a new "Streaming"-tab besides "Layout"), right-click "Master"→"start" to start the server.
You can provide your workspace name (you might want to rename it before you start thes streaming), otherwise it defaults to workspace0
The default Gephi-URL is http://localhost:8080, resulting in http://localhost:8080/workspace0?operation=updateGraph
You can also configure it in conf/neo4j.conf
via apoc.gephi.url=url
or apoc.gephi.<key>.url=url
Example
match path = (:Person)-[:ACTED_IN]->(:Movie)
WITH path LIMIT 1000
with collect(path) as paths
call apoc.gephi.add(null,'workspace0', paths) yield nodes, relationships, time
return nodes, relationships, time

Format
We send all nodes and relationships of the passed in data convert into individual Gephi-Streaming JSON fragements, separated by \r\n
.
{"an":{"123":{"TYPE":"Person:Actor","label":"Tom Hanks", x:333,y:222,r:0.1,g:0.3,b:0.5}}}\r\n
{"an":{"345":{"TYPE":"Movie","label":"Forrest Gump", x:234,y:122,r:0.2,g:0.2,b:0.7}}}\r\n
{"ae":{"3344":{"TYPE":"ACTED_IN","label":"Tom Hanks",source:"123",target:"345","directed":true,r:0.1,g:0.3,b:0.5}}}
Specifics Details
Gephi doesn’t render the graph data unless you also provide x,y coordinates in the payload, so we just send random ones within a 1000x1000 grid.
We also generate colors per label combination and relationship-type, both of which are also transferred as TYPE
property.
ElasticSearch Integration
|
elastic search statistics |
|
perform a GET operation |
|
perform a SEARCH operation |
|
perform a raw GET operation |
|
perform a raw POST operation |
|
perform a POST operation |
|
perform a PUT operation |
Example
call apoc.es.post("localhost","tweets","users","1",null,{name:"Chris"})
call apoc.es.get("localhost","tweets","users","1",null,null)
call apoc.es.stats("localhost")

General Structure and Parameters
call apoc.es.post(host-or-port,index-or-null,type-or-null,id-or-null,query-or-null,payload-or-null) yield value
// GET/PUT/POST url/index/type/id?query -d payload
host or port parameter
The parameter can be a direct host or url, or an entry to be lookup up in neo4j.conf
-
host
-
host:port
-
lookup via key to apoc.es.<key>.url
-
lookup via key apoc.es.<key>.host
-
lookup apoc.es.url
-
lookup apoc.es.host
index parameter
Main ES index, will be sent directly, if null then "_all" multiple indexes can be separated by comma in the string.
type parameter
Document type, will be sent directly, if null then "_all" multiple types can be separated by comma in the string.
id parameter
Document id, will be left off when null.
query parameter
Query can be a map which is turned into a query string, a direct string or null then it is left off.
payload parameter
Payload can be a map which will be turned into a json payload or a string which will be sent directly or null.
Results
Results are stream of map in value.
Load XML
Load XML Introduction
Many existing (enterprise) applications, endpoints and files use XML as data exchange format.
To make these datastructures available to Cypher, you can use apoc.load.xml(Simple)
.
It takes a file or http URL and parses the XML into a map datastructure.
While apoc.load.xml
has a more verbose format, it keeps the ordering of elements.
While apoc.load.xmlSimple
provides a more compact representation that makes it easier to process.
See the following usage-examples for the procedures.
Example File
"How do you access XML doc attributes in children fields ?"
(Thanks Nicolas Rouyer)
For example, if my XML file is the example book.xml provided by Microsoft.
<?xml version="1.0"?>
<catalog>
<book id="bk101">
<author>Gambardella, Matthew</author>
<title>XML Developer's Guide</title>
<genre>Computer</genre>
<price>44.95</price>
<publish_date>2000-10-01</publish_date>
<description>An in-depth look at creating applications
with XML.</description>
</book>
<book id="bk102">
<author>Ralls, Kim</author>
<title>Midnight Rain</title>
<genre>Fantasy</genre>
<price>5.95</price>
<publish_date>2000-12-16</publish_date>
<description>A former architect battles corporate zombies,
...
We have the file here, on GitHub.
Simple XML Format
In a simpler XML representation, each type of children gets it’s own entry within the parent map. The element-type as key is prefixed with "_" to prevent collisions with attributes.
If there is a single element, then the entry will just have that element as value, not a collection. If there is more than one element there will be a list of values.
Each child will still have its _type
field to discern them.
Here is the example file from above loaded with apoc.load.xmlSimple
call apoc.load.xmlSimple("https://raw.githubusercontent.com/neo4j-contrib/neo4j-apoc-procedures/master/src/test/resources/books.xml")
{_type: "catalog", _book: [
{_type: "book", id: "bk101",
_author: [{_type: "author", _text: "Gambardella, Matthew"},{_type: author, _text: "Arciniegas, Fabio"}],
_title: {_type: "title", _text: "XML Developer's Guide"},
_genre: {_type: "genre", _text: "Computer"},
_price: {_type: "price", _text: "44.95"},
_publish_date: {_type: "publish_date", _text: "2000-10-01"},
_description: {_type: description, _text: An in-depth look at creating applications ....
Load XML and Introspect
Let’s just load it and see what it looks like.
It’s returned as value map with nested _type
and _children
fields, per group of elements.
Attributes are turned into map-entries.
And each element into their own little map with _type
, attributes and _children
if applicable.
call apoc.load.xml("https://raw.githubusercontent.com/neo4j-contrib/neo4j-apoc-procedures/master/src/test/resources/books.xml")
{_type: catalog, _children: [
{_type: book, id: bk101, _children: [
{_type: author, _text: Gambardella, Matthew},
{_type: title, _text: XML Developer's Guide},
{_type: genre, _text: Computer},
{_type: price, _text: 44.95},
{_type: publish_date, _text: 2000-10-01},
{_type: description, _text: An in-depth look at creating applications ....
For each book, how do I access book id ?
You can access attributes per element directly.
call apoc.load.xml("https://raw.githubusercontent.com/neo4j-contrib/neo4j-apoc-procedures/master/src/test/resources/books.xml") yield value as catalog
UNWIND catalog._children as book
RETURN book.id
╒═══════╕ │book.id│ ╞═══════╡ │bk101 │ ├───────┤ │bk102 │
For each book, how do I access book author and title ?
Filter into collection
You have to filter over the sub-elements in the _childrens
array in this case.
call apoc.load.xml("https://raw.githubusercontent.com/neo4j-contrib/neo4j-apoc-procedures/master/src/test/resources/books.xml") yield value as catalog
UNWIND catalog._children as book
RETURN book.id, [attr IN book._children WHERE attr._type IN ['author','title'] | [attr._type, attr._text]] as pairs
╒═══════╤════════════════════════════════════════════════════════════════════════╕ │book.id│pairs │ ╞═══════╪════════════════════════════════════════════════════════════════════════╡ │bk101 │[[author, Gambardella, Matthew], [title, XML Developer's Guide]] │ ├───────┼────────────────────────────────────────────────────────────────────────┤ │bk102 │[[author, Ralls, Kim], [title, Midnight Rain]] │
How do I return collection elements?
This is not too nice, we could also just have returned the values and then grabbed them out of the list, but that relies on element-order.
call apoc.load.xml("https://raw.githubusercontent.com/neo4j-contrib/neo4j-apoc-procedures/master/src/test/resources/books.xml") yield value as catalog
UNWIND catalog._children as book
WITH book.id as id, [attr IN book._children WHERE attr._type IN ['author','title'] | attr._text] as pairs
RETURN id, pairs[0] as author, pairs[1] as title
╒═════╤════════════════════╤══════════════════════════════╕ │id │author │title │ ╞═════╪════════════════════╪══════════════════════════════╡ │bk101│Gambardella, Matthew│XML Developer's Guide │ ├─────┼────────────────────┼──────────────────────────────┤ │bk102│Ralls, Kim │Midnight Rain │
Extracting Datastructures
Turn Pairs into Map
So better is to turn them into a map with apoc.map.fromPairs
call apoc.load.xml("https://raw.githubusercontent.com/neo4j-contrib/neo4j-apoc-procedures/master/src/test/resources/books.xml") yield value as catalog
UNWIND catalog._children as book
WITH book.id as id, [attr IN book._children WHERE attr._type IN ['author','title'] | [attr._type, attr._text]] as pairs
CALL apoc.map.fromPairs(pairs) yield value
RETURN id, value
╒═════╤════════════════════════════════════════════════════════════════════╕ │id │value │ ╞═════╪════════════════════════════════════════════════════════════════════╡ │bk101│{author: Gambardella, Matthew, title: XML Developer's Guide} │ ├─────┼────────────────────────────────────────────────────────────────────┤ │bk102│{author: Ralls, Kim, title: Midnight Rain} │ ├─────┼────────────────────────────────────────────────────────────────────┤ │bk103│{author: Corets, Eva, title: Maeve Ascendant} │
Return individual Columns
And now we can cleanly access the attributes from the map.
call apoc.load.xml("https://raw.githubusercontent.com/neo4j-contrib/neo4j-apoc-procedures/master/src/test/resources/books.xml") yield value as catalog
UNWIND catalog._children as book
WITH book.id as id, [attr IN book._children WHERE attr._type IN ['author','title'] | [attr._type, attr._text]] as pairs
CALL apoc.map.fromPairs(pairs) yield value
RETURN id, value.author, value.title
╒═════╤════════════════════╤══════════════════════════════╕ │id │value.author │value.title │ ╞═════╪════════════════════╪══════════════════════════════╡ │bk101│Gambardella, Matthew│XML Developer's Guide │ ├─────┼────────────────────┼──────────────────────────────┤ │bk102│Ralls, Kim │Midnight Rain │ ├─────┼────────────────────┼──────────────────────────────┤ │bk103│Corets, Eva │Maeve Ascendant │
Graph Refactorings
Graph Refactoring Procedures
These procedures help refactor the structure of your graph. This is helpful when you need to change your data model or for cleaning up data that was imported from an external source.
Normalize boolean properties
Given raw data boolean properties are often represented by placeholder values. This procedure turns them into boolean properties.
Will be true if "Y", YES"; false if "N", "NO"; null otherwise:
MATCH (n)
CALL apoc.refactor.normalizeAsBoolean(n, "prop", ["Y", "YES"], ["N", NO"])
RETURN n.prop
Categorization
Categorize replaces string property values on nodes with relationship to a unique category node for that property value.
This example will turn all n.color properties into :HAS_ATTRIBUTE relationships to :Color nodes with a matching .colour property.
CALL apoc.refactor.categorize('color','HAS_ATTRIBUTE',true,'Color','colour',['popularity'],1)
Additionally, it will also copy over the first 'popularity' property value encountered on any node n for each newly created :Color node and remove any occurrences of that property value on nodes with the same 'Color'.
Using Cypher and APOC to move a property value to a label
You can use the procedure apoc.create.addLabels
to move a property to a label with Cypher as follows
CREATE (:Movie {title: 'A Few Good Men', genre: 'Drama'})
MATCH (n:Movie)
CALL apoc.create.addLabels( id(n), [ n.genre ] ) YIELD node
REMOVE node.studio
RETURN node
Cypher Operations
Running Cypher fragments
We can use Cypher as safe, graph-aware, partially compiled scripting language within APOC.
Cypher Execution
|
executes reading fragment with the given parameters |
|
runs each statement in the file, all semicolon separated - currently no schema operations |
|
runs each semicolon separated statement and returns summary - currently no schema operations |
|
executes fragment in parallel batches with the list segments being assigned to _ |
|
executes writing fragment with the given parameters |
|
abort statement after timeout millis if not finished |
Example: Fast Node-Counts by Label
Counts by label are quickly provided by the counts-store, but only if they are the the single thing in the query, like
MATCH (:Person) RETURN count(*);
It also works to combine several with UNION ALL
, but not via WITH
MATCH (:Person) WITH count(*) as people
MATCH (:Movie) RETURN people, count(*) as movies;
MATCH (:Person) RETURN count(*)
UNION ALL
MATCH (:Movie) RETURN count(*);
But with apoc.cypher.run
we can construct the statement and run each of them individually, so it completes in a few ms.
call db.labels() yield label
call apoc.cypher.run("match (:`"+label+"`) return count(*) as count", null) yield value
return label, value.count as count
You can use a similar approach to get the property-keys per label:
CALL db.labels() yield label
call apoc.cypher.run("MATCH (n:`"+label+"`) RETURN keys(n) as keys LIMIT 1",null) yield value
RETURN label, value.keys as keys
running a cypher statement timeboxed
There’s a way to terminate a cypher statement if it takes longer than a given threshold. Consider an expensive statement calculating cross product of shortestpaths for each pair of nodes:
call apoc.cypher.runTimeboxed("match (n),(m) match p=shortestPath((n)-[*]-(m)) return p", null, 10000) yield value
return value.p
Job management and periodic execution
Introduction asynchronous transactional execution
Note
|
this document is work in progress |
Cypher is great for querying graphs and importing and updating graph structures.
While during imports you can use PERIODIC COMMIT
to control transaction sizes in memory,
for other graph refactorings it’s not that easy to commit transactions regularly to free memory for new update state.
Also sometimes you want to schedule execution of Cypher statements to run regularly in the background or asynchronously ("fire & forget").
The apoc.periodic.*
procedures provide such capabilities.
apoc.periodic.iterate
With apoc.periodic.iterate
you provide 2 statements, the first outer statement is providing a stream of values to be processed.
The second, inner statement processes one element at a time or with iterateList:true
the whole batch at a time.
The results of the outer statement are passed into the inner statement as parameters, they are automatically made available with their names.
param | default | description |
---|---|---|
batchSize |
1000 |
that many inner statements are run within a single tx params: {_count, _batch} |
parallel |
false |
run inner statement in parallel, note that statements might deadlock |
retries |
0 |
if the inner statement fails with an error, sleep 100ms and retry until retries-count is reached, param {_retry} |
iterateList |
false |
the inner statement is only executed once but the whole batchSize list is passed in as parameter {_batch} |
params |
{} |
externally passed in map of params |
Note
|
We plan to make iterateList:true the default in upcoming releases, due to the automatic UNWINDing and providing of nested results as variables,
most queries should continue work.
|
So if you were to add an :Actor
label to several million :Person
nodes, you would run:
CALL apoc.periodic.iterate(
"MATCH (p:Person) WHERE (p)-[:ACTED_IN]->() RETURN p",
"SET p:Actor", {batchSize:10000, parallel:true})
Which would take 10k people from the stream and update them in a single transaction, executing the second statement for each person.
Those executions can happen in parallel as updating node-labels or properties doesn’t conflict.
If you do more complex operations like updating or removing relationships, either don’t use parallel OR make sure that you batch the work in a way that each subgraph of data is updated in one operation, e.g. by transferring the root objects.
If you attempt complex operations, try to use e.g. retries:3
to retry failed operations.
CALL apoc.periodic.iterate(
"MATCH (o:Order) WHERE o.date > '2016-10-13' RETURN o",
"MATCH (o)-[:HAS_ITEM]->(i) WITH o, sum(i.value) as value SET o.value = value", {batchSize:100, parallel:true})
CALL apoc.periodic.iterate(
"MATCH (o:Order) WHERE o.date > '2016-10-13' RETURN o",
"MATCH (o)-[:HAS_ITEM]->(i) WITH o, sum(i.value) as value SET o.value = value", {batchSize:100, iterateList:true, parallel:true})
The stream of other data can also come from another source, like a different database, CSV or JSON file.
apoc.periodic.commit
Especially for graph processing it is useful to run a query repeatedly in separate transactions until it doesn’t process and generates any results anymore. So you can iterate in batches over elements that don’t fulfill a condition and update them so that they do afterwards.
The query is executed repatedly in separate transactions until it returns 0.
call apoc.periodic.commit("
match (user:User) WHERE exists( user.city )
with user limit {limit}
MERGE (city:City {name:user.city})
MERGE (user)-[:LIVES_IN]->(city)
REMOVE user.city
RETURN count(*)
",{limit:10000})
+=======+==========+ |updates|executions| +=======+==========+ |2000000|200 | +-------+----------+
Further Functions
|
repeats an batch update statement until it returns 0, this procedure is blocking |
|
list all jobs |
|
submit a one-off background statement |
|
submit a repeatedly-called background statement |
|
submit a repeatedly-called background statement until it returns 0 |
|
iterate over first statement and apply action statement with given transaction batch size. Returns to numeric values holding the number of batches and the number of total processed rows. E.g. |
|
run the second statement for each item returned by the first statement. Returns number of batches and total processed rows |
-
there are also static methods
Jobs.submit
, andJobs.schedule
to be used from other procedures -
jobs list is checked / cleared every 10s for finished jobs
name
property of each person to lastname
CALL apoc.periodic.rock_n_roll('match (p:Person) return id(p) as id_p', 'MATCH (p) where id(p)={id_p} SET p.lastname =p.name', 20000)